Tutorial: Brd4 bindings for Male/Female mouse bulk calling cards data.#
We will analyze bulk calling cards datasets from Kfoury et al., PNAS. (2021), which can be downloaded from GEO. These experiments were designed to study differences in Brd4 binding between male and female cells in a genetically engineered cellular model of glioblastoma (GBM). These nearly-isogenic cells were derived from murine neocortical postnatal day 1 (p1) astrocytes (male and female GBM astrocytes) and were engineered to contain a combined loss of neurofibromin (NF1) and p53 function. These cells were previously shown to display sex differences in important GBM phenotypes in vivo, including proliferation, clonogenic stem-like frequency, in vivo tumorigenesis, cell cycle regulation, gene expression, and chemotherapy response, that mimic those observed in GBM patients. For more details see [Kfoury et al., PNAS. (2021)].
In this tutorial, we will call peaks, annotate these peaks, perform differential peak analysis, and pair differentially bound genomic regions with nearby genes that are also differentially expressed between the sexes. There are 668525 insertions in female qbed data and 613728 insertions in male qbed data. For bulk RNA-seq data, there are 6 samples in total: 3 female samples and 3 male samples. It creates a group by peak anndata for calling cards data. If you want to create a replicate by peak anndata only, please check Github
[1]:
import pycallingcards as cc
import numpy as np
import pandas as pd
import scanpy as sc
from matplotlib import pyplot as plt
plt.rcParams['figure.dpi'] = 150
We start by reading qbed data. In these data, each row provides information about one Brd4-directed insertion organized into columns to indicate the chromosome, start point, end point, number of reads supporting the insertion, and the orientation and barcode for each insertion. For example, in the file displayed below, the first row tells us that the first insertion occured into a TTAA on Chromosome 1, whose coordinates start location 3478112 and end at 3478115. There was 1 read supporting this insertion and the transposon is orientated so that the plus strand of the transposon is on the plus strand of the genome. The transposon barcode is TTTGTCCAA. We add another column indecating its respected group.
Use cc.rd.read_qbed(filename)
to load your own qbed data.
[2]:
Female_Brd4 = cc.datasets.mouse_brd4_data(data = "Female_Brd4")
Female_Brd4['group'] = "Female_Brd4"
Female_Brd4
#load female CC data
[2]:
Chr | Start | End | Reads | Direction | Barcodes | group | |
---|---|---|---|---|---|---|---|
0 | chr1 | 3478111 | 3478115 | 1 | + | TTTGTCCAA | Female_Brd4 |
1 | chr1 | 3481743 | 3481747 | 1 | + | TATGTACAA | Female_Brd4 |
2 | chr1 | 3481957 | 3481961 | 1 | - | TATGTACAA | Female_Brd4 |
3 | chr1 | 3493161 | 3493165 | 16 | + | TATGTACAA | Female_Brd4 |
4 | chr1 | 3493702 | 3493706 | 1 | + | CGTTACACA | Female_Brd4 |
... | ... | ... | ... | ... | ... | ... | ... |
1290214 | chrY | 90802841 | 90802845 | 1 | - | CGTTACACA | Female_Brd4 |
1290215 | chrY | 90803579 | 90803583 | 1 | - | TATGTACAA | Female_Brd4 |
1290216 | chrY | 90803579 | 90803583 | 1 | - | TGTCGTGCA | Female_Brd4 |
1290217 | chrY | 90804429 | 90804433 | 1 | - | TATACTCTA | Female_Brd4 |
1290218 | chrY | 90805130 | 90805134 | 1 | - | GACGGCTCC | Female_Brd4 |
1290219 rows × 7 columns
[3]:
Male_Brd4 = cc.datasets.mouse_brd4_data(data = "Male_Brd4")
Male_Brd4['group'] = "Male_Brd4"
Male_Brd4
#load male CC data
[3]:
Chr | Start | End | Reads | Direction | Barcodes | group | |
---|---|---|---|---|---|---|---|
0 | chr1 | 3493161 | 3493165 | 1 | - | GAGGTACAG | Male_Brd4 |
1 | chr1 | 3493275 | 3493279 | 1 | + | TACTTTCCG | Male_Brd4 |
2 | chr1 | 3493341 | 3493345 | 2 | + | AGCACAGTG | Male_Brd4 |
3 | chr1 | 3493341 | 3493345 | 10 | + | TGTCCATTG | Male_Brd4 |
4 | chr1 | 3493341 | 3493345 | 8 | + | ATGAAAGCA | Male_Brd4 |
... | ... | ... | ... | ... | ... | ... | ... |
1185697 | chrY | 90803733 | 90803737 | 13 | - | TACTTTCCG | Male_Brd4 |
1185698 | chrY | 90803733 | 90803737 | 9 | - | CACGGCGGA | Male_Brd4 |
1185699 | chrY | 90803733 | 90803737 | 17 | - | GATCTTATG | Male_Brd4 |
1185700 | chrY | 90803774 | 90803778 | 4 | - | ATGAAAGCA | Male_Brd4 |
1185701 | chrY | 90806562 | 90806566 | 1 | - | AGCACAGTG | Male_Brd4 |
1185702 rows × 7 columns
Since we are interested in differential peak calling, we now combine the two datasets to call peaks on the joint dataset:
[4]:
Brd4 = cc.rd.combine_qbed([Female_Brd4, Male_Brd4])
Brd4
[4]:
Chr | Start | End | Reads | Direction | Barcodes | group | |
---|---|---|---|---|---|---|---|
0 | chr1 | 3478111 | 3478115 | 1 | + | TTTGTCCAA | Female_Brd4 |
1 | chr1 | 3481743 | 3481747 | 1 | + | TATGTACAA | Female_Brd4 |
2 | chr1 | 3481957 | 3481961 | 1 | - | TATGTACAA | Female_Brd4 |
3 | chr1 | 3493161 | 3493165 | 16 | + | TATGTACAA | Female_Brd4 |
4 | chr1 | 3493161 | 3493165 | 1 | - | GAGGTACAG | Male_Brd4 |
... | ... | ... | ... | ... | ... | ... | ... |
2475916 | chrY | 90803733 | 90803737 | 17 | - | GATCTTATG | Male_Brd4 |
2475917 | chrY | 90803774 | 90803778 | 4 | - | ATGAAAGCA | Male_Brd4 |
2475918 | chrY | 90804429 | 90804433 | 1 | - | TATACTCTA | Female_Brd4 |
2475919 | chrY | 90805130 | 90805134 | 1 | - | GACGGCTCC | Female_Brd4 |
2475920 | chrY | 90806562 | 90806566 | 1 | - | AGCACAGTG | Male_Brd4 |
2475921 rows × 7 columns
Optional: filter the insertions with reads greater than 2. Because we use strict quality control on read insertions, even insertions supported by 1 read are likely to be bona fide.
[5]:
Brd4 = Brd4[Brd4['Reads'] > 2]
Brd4
[5]:
Chr | Start | End | Reads | Direction | Barcodes | group | |
---|---|---|---|---|---|---|---|
3 | chr1 | 3493161 | 3493165 | 16 | + | TATGTACAA | Female_Brd4 |
7 | chr1 | 3493341 | 3493345 | 10 | + | TGTCCATTG | Male_Brd4 |
8 | chr1 | 3493341 | 3493345 | 8 | + | ATGAAAGCA | Male_Brd4 |
10 | chr1 | 3493643 | 3493647 | 3 | + | TCTACTGCC | Male_Brd4 |
17 | chr1 | 3514553 | 3514557 | 7 | + | TACTTTCCG | Male_Brd4 |
... | ... | ... | ... | ... | ... | ... | ... |
2475905 | chrY | 42512546 | 42512550 | 3 | - | ACGCGCTGT | Male_Brd4 |
2475914 | chrY | 90803733 | 90803737 | 13 | - | TACTTTCCG | Male_Brd4 |
2475915 | chrY | 90803733 | 90803737 | 9 | - | CACGGCGGA | Male_Brd4 |
2475916 | chrY | 90803733 | 90803737 | 17 | - | GATCTTATG | Male_Brd4 |
2475917 | chrY | 90803774 | 90803778 | 4 | - | ATGAAAGCA | Male_Brd4 |
1229664 rows × 7 columns
Because insertions are discrete, we now need to call peaks to deduce potential binding sites. Three different methods (CCcaller, MACCs, Blockify) are available along with three different species (hg38, mm10, sacCer3).
In this setting, we use CCcaller in mouse(‘mm10’) data. maxbetween is the most important parameter for CCcaller. It controls the maximum distance between two nearby insertions, or in another words, the minimum distance between two peaks. We recommend using a value between 800-1200 to set maxbetween. pvalue_cutoff is also an important parameter and a number 0.0001-0.01 is strongly advised. The setting of pseudocounts is largely influenced by library size. For the first time of trial, it can be adjusted to \(10^{6}-10^{-5} \times\) the number of insertions.
[6]:
peak_data = cc.pp.call_peaks(Brd4, method = "CCcaller", reference = "mm10", pvalue_cutoff = 0.01,
maxbetween = 1100, lam_win_size = 1000000, pseudocounts = 0.1,
record = True, save = "peak.bed")
peak_data
For the CCcaller method without background, [expdata, reference, pvalue_cutoff, lam_win_size, pseudocounts, minlen, extend, maxbetween, test_method, min_insertions, record] would be utilized.
100%|██████████| 21/21 [00:21<00:00, 1.00s/it]
[6]:
Chr | Start | End | Experiment Insertions | Reference Insertions | Expected Insertions | pvalue | pvalue_adj | |
---|---|---|---|---|---|---|---|---|
0 | chr1 | 4196775 | 4198714 | 16 | 12 | 0.142389 | 0.000000e+00 | 0.000000e+00 |
1 | chr1 | 4785068 | 4786551 | 17 | 15 | 0.572576 | 0.000000e+00 | 0.000000e+00 |
2 | chr1 | 4806673 | 4807730 | 21 | 12 | 0.477937 | 0.000000e+00 | 0.000000e+00 |
3 | chr1 | 4856928 | 4862019 | 104 | 44 | 1.490080 | 0.000000e+00 | 0.000000e+00 |
4 | chr1 | 4912814 | 4916593 | 7 | 22 | 0.791756 | 1.900897e-06 | 1.024407e-04 |
... | ... | ... | ... | ... | ... | ... | ... | ... |
24546 | chrX | 169936677 | 169937745 | 7 | 8 | 1.189526 | 3.479200e-05 | 1.673495e-03 |
24547 | chrY | 897430 | 899700 | 11 | 24 | 0.528165 | 6.046275e-13 | 5.162843e-11 |
24548 | chrY | 1009018 | 1011799 | 27 | 25 | 0.489073 | 0.000000e+00 | 0.000000e+00 |
24549 | chrY | 1243715 | 1246316 | 24 | 29 | 0.504932 | 0.000000e+00 | 0.000000e+00 |
24550 | chrY | 1282482 | 1287504 | 19 | 60 | 0.945958 | 0.000000e+00 | 0.000000e+00 |
24551 rows × 8 columns
In order to tune parameters for peak calling, we advise looking at the data and evaluating the validity of the called peaks. The default settings are what we recommend, but for some TFs adjacent peaks may be merged that should not be, or, alternatively, peaks that should be joined may be called separately.
Below, we plot the combined calling card data in males and females for a region in chromosome 1. The top track displays insertion locations and their read counts. Each dot is an insertion and the height is log(reads+1). The middle track plots the insertion density. The thrid track represents the reference genes and peaks. Finally, the last track represents peak calls. Below you can see that regions with high densities of insertions are accurately called as Brd4 binding sites.
[7]:
cc.pl.draw_area("chr1", 4856929, 4863861, 100000, peak_data, Brd4, "mm10", font_size = 2,
plotsize = [1,1,5],
figsize = (30,10), peak_line = 2, save = False, example_length = 5000)
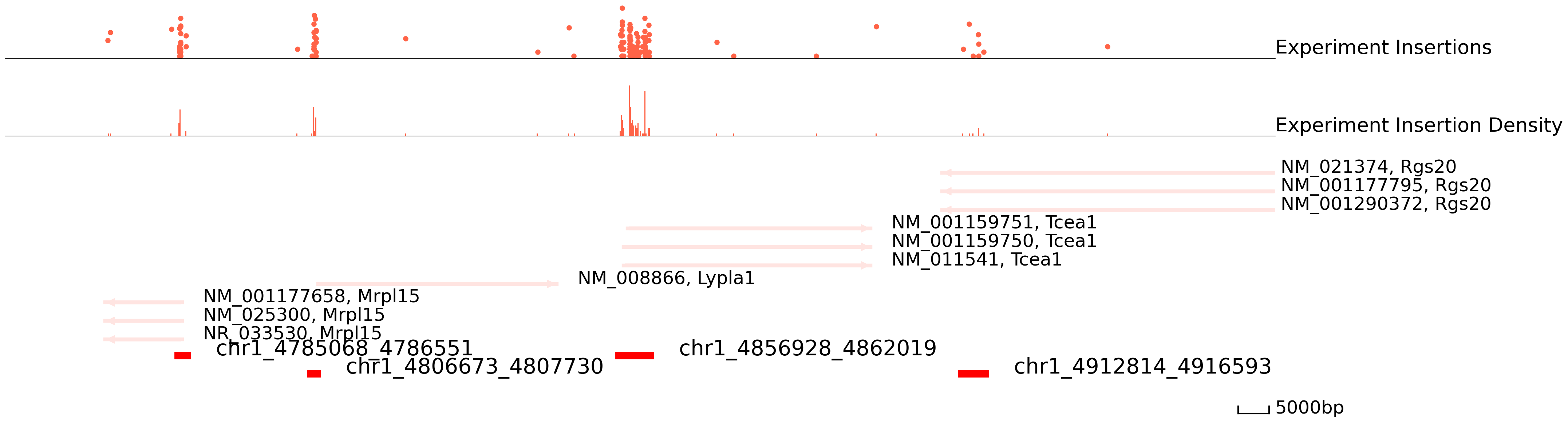
[8]:
cc.pl.draw_area("chr2", 6136575, 6144043, 75000, peak_data, Brd4, "mm10", font_size = 2, plotsize = [1,1,5],
figsize = (30,8), peak_line = 1, save = False, example_length = 5000)
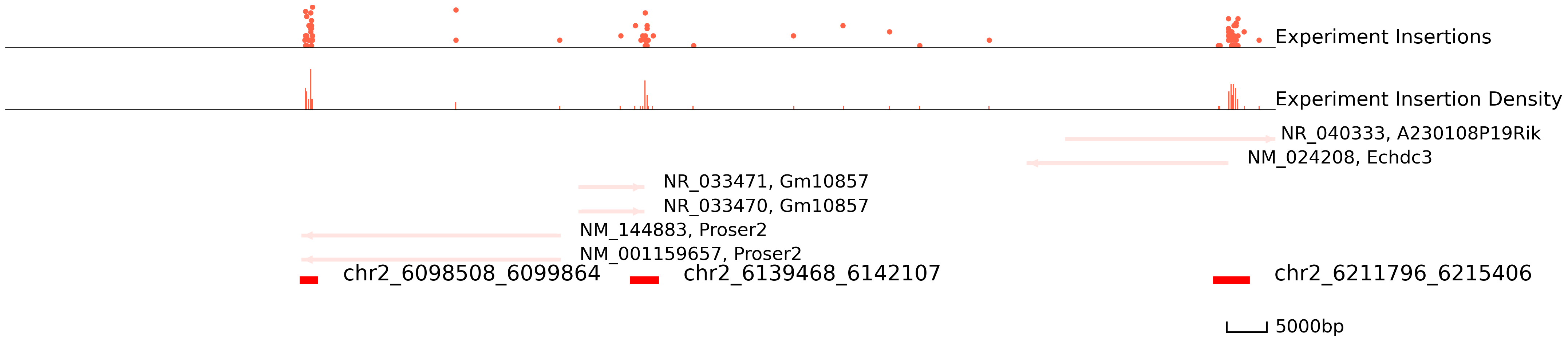
We can also visualize our data directly in the WashU Epigenome Browser. This can be useful for overlaying your data with other published datasets. Notice that this link only valid for 24hrs, so please rerun it if you want to use it.
[9]:
qbed = {"Brd4": Brd4}
bed = {"peak": peak_data}
cc.pl.WashU_browser_url(qbed = qbed, bed = bed, genome = 'mm10')
All qbed addressed
All bed addressed
Uploading files
Please click the following link to see the data on WashU Epigenome Browser directly.
https://epigenomegateway.wustl.edu/browser/?genome=mm10&hub=https://companion.epigenomegateway.org//task/544b112245ab68df385f75da9d4bfd8b/output//datahub.json
Pycallingcards can be used to visual peak locations acorss the genome to see if the distribution of peaks is unbiased and all chromosomes are represented.
[10]:
cc.pl.whole_peaks(peak_data, reference = "mm10", linewidth = 1)
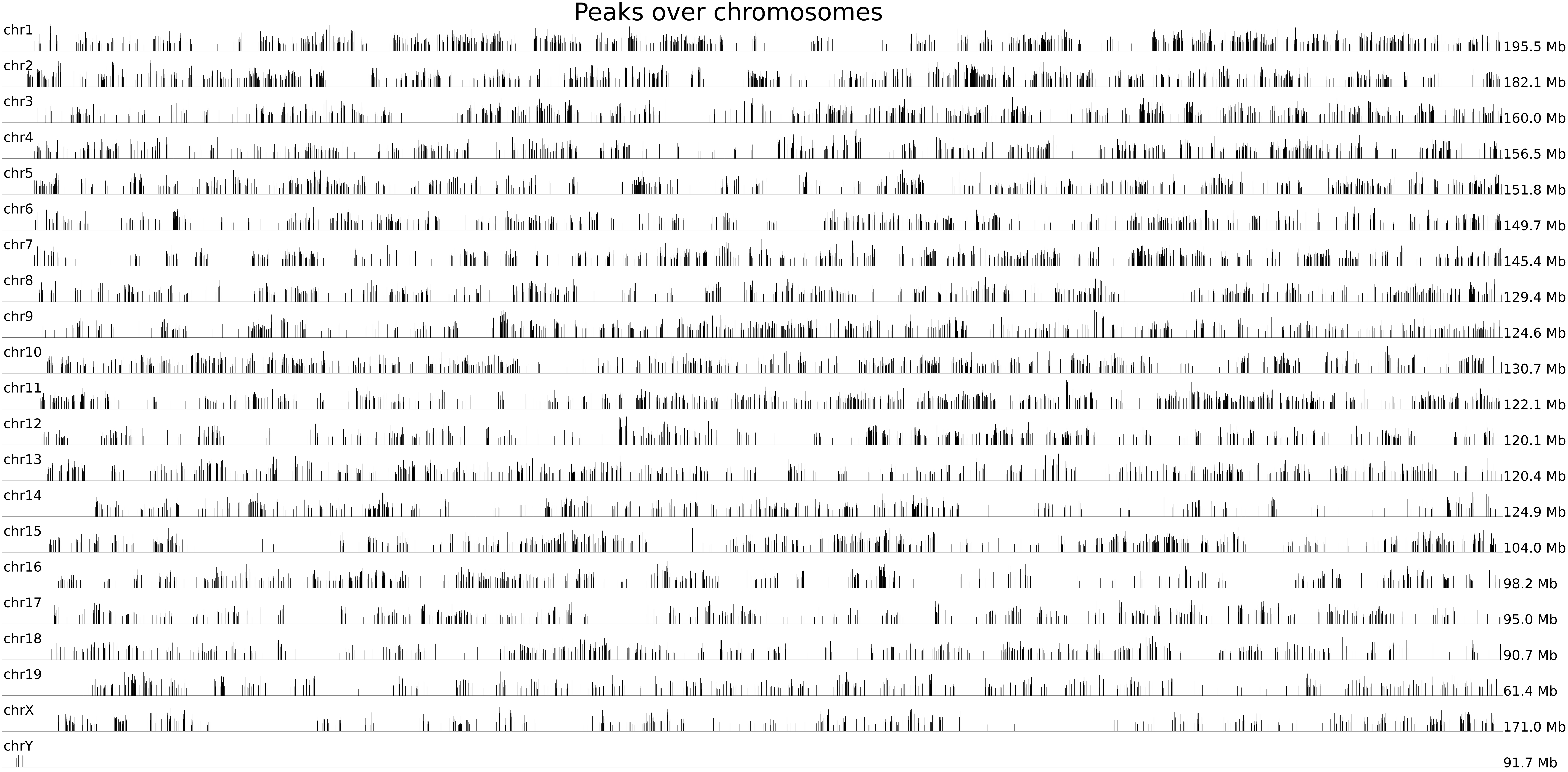
For differential peak calling, we recommend first combining the data and calling peaks together (and then splitting the data and looking for enrichments under the jointly called peaks). However, it is often of interest to call peaks in each sample to analyze them separately or to then merge these peak calls using pybedtools. Below is the code to do this:
import pybedtools
peak_data1 = cc.pp.call_peaks(Female_Brd4, method = "CCcaller",
reference = "mm10", pvalue_cutoffbg = 0.1,
maxbetween = 2000, pvalue_cutoffTTAA = 0.001,
lam_win_size = 1000000, pseudocounts = 0.1, record = True)
peak_data2 = cc.pp.call_peaks(Male_Brd4, method = "CCcaller",
reference = "mm10", pvalue_cutoffbg = 0.1,
maxbetween = 2000, pvalue_cutoffTTAA = 0.001,
lam_win_size = 1000000, pseudocounts = 0.1, record = True)
peak = cc.rd.combine_qbed([peak_data1, peak_data2])
peak = pybedtools.BedTool.from_dataframe(peak).merge().to_dataframe()
peak_data = peak.rename(columns={"chrom":"Chr", "start":"Start", "end":"End"})
In the next step, we annote each peak to annotate using bedtools and pybedtools. Make sure they are all previously installed before using.
[11]:
peak_annotation = cc.pp.annotation(peak_data, reference = "mm10")
peak_annotation = cc.pp.combine_annotation(peak_data, peak_annotation)
peak_annotation
In the bedtools method, we would use bedtools in the default path. Set bedtools path by 'bedtools_path' if needed.
[11]:
Chr | Start | End | Experiment Insertions | Reference Insertions | Expected Insertions | pvalue | pvalue_adj | Nearest Refseq1 | Gene Name1 | Direction1 | Distance1 | Nearest Refseq2 | Gene Name2 | Direction2 | Distance2 | |
---|---|---|---|---|---|---|---|---|---|---|---|---|---|---|---|---|
0 | chr1 | 4196775 | 4198714 | 16 | 12 | 0.142389 | 0.000000e+00 | 0.000000e+00 | NM_001195662 | Rp1 | - | 92132 | NM_011283 | Rp1 | - | 144793 |
1 | chr1 | 4785068 | 4786551 | 17 | 15 | 0.572576 | 0.000000e+00 | 0.000000e+00 | NR_033530 | Mrpl15 | - | 0 | NM_008866 | Lypla1 | + | 21342 |
2 | chr1 | 4806673 | 4807730 | 21 | 12 | 0.477937 | 0.000000e+00 | 0.000000e+00 | NM_008866 | Lypla1 | + | 163 | NR_033530 | Mrpl15 | - | -20948 |
3 | chr1 | 4856928 | 4862019 | 104 | 44 | 1.490080 | 0.000000e+00 | 0.000000e+00 | NM_011541 | Tcea1 | + | 0 | NM_008866 | Lypla1 | + | -10194 |
4 | chr1 | 4912814 | 4916593 | 7 | 22 | 0.791756 | 1.900897e-06 | 1.024407e-04 | NM_001290372 | Rgs20 | - | 0 | NM_011541 | Tcea1 | + | -14906 |
... | ... | ... | ... | ... | ... | ... | ... | ... | ... | ... | ... | ... | ... | ... | ... | ... |
24546 | chrX | 169936677 | 169937745 | 7 | 8 | 1.189526 | 3.479200e-05 | 1.673495e-03 | NM_010797 | Mid1 | + | 0 | NR_029457 | G530011O06Rik | - | 37298 |
24547 | chrY | 897430 | 899700 | 11 | 24 | 0.528165 | 6.046275e-13 | 5.162843e-11 | NM_011419 | Kdm5d | + | 0 | NM_011667 | Uba1y | + | -53207 |
24548 | chrY | 1009018 | 1011799 | 27 | 25 | 0.489073 | 0.000000e+00 | 0.000000e+00 | NM_012011 | Eif2s3y | + | 0 | NR_027507 | Tspy-ps | - | 43965 |
24549 | chrY | 1243715 | 1246316 | 24 | 29 | 0.504932 | 0.000000e+00 | 0.000000e+00 | NM_009484 | Uty | - | 0 | NM_012008 | Ddx3y | - | 14399 |
24550 | chrY | 1282482 | 1287504 | 19 | 60 | 0.945958 | 0.000000e+00 | 0.000000e+00 | NM_012008 | Ddx3y | - | 0 | NM_148943 | Usp9y | - | 11457 |
24551 rows × 16 columns
Use qbed data, peak data, and barcode data to make a group by peak Anndata object.
[12]:
adata_cc = cc.pp.make_Anndata(Brd4, peak_annotation, ["Female_Brd4", "Male_Brd4"], key = 'group')
adata_cc
100%|██████████| 21/21 [00:07<00:00, 2.64it/s]
[12]:
AnnData object with n_obs × n_vars = 2 × 24551
var: 'Chr', 'Start', 'End', 'Experiment Insertions', 'Reference Insertions', 'Expected Insertions', 'pvalue', 'pvalue_adj', 'Nearest Refseq1', 'Gene Name1', 'Direction1', 'Distance1', 'Nearest Refseq2', 'Gene Name2', 'Direction2', 'Distance2'
[13]:
adata_cc.obs
[13]:
Index |
---|
Female_Brd4 |
Male_Brd4 |
[14]:
adata_cc
[14]:
AnnData object with n_obs × n_vars = 2 × 24551
var: 'Chr', 'Start', 'End', 'Experiment Insertions', 'Reference Insertions', 'Expected Insertions', 'pvalue', 'pvalue_adj', 'Nearest Refseq1', 'Gene Name1', 'Direction1', 'Distance1', 'Nearest Refseq2', 'Gene Name2', 'Direction2', 'Distance2'
[15]:
adata_cc = cc.tl.liftover(adata_cc)
adata_cc
100%|██████████| 24551/24551 [04:44<00:00, 86.27it/s]
[15]:
AnnData object with n_obs × n_vars = 2 × 24551
var: 'Chr', 'Start', 'End', 'Experiment Insertions', 'Reference Insertions', 'Expected Insertions', 'pvalue', 'pvalue_adj', 'Nearest Refseq1', 'Gene Name1', 'Direction1', 'Distance1', 'Nearest Refseq2', 'Gene Name2', 'Direction2', 'Distance2', 'Chr_liftover', 'Start_liftover', 'End_liftover'
Differential peak analysis will find out the significant binding for each group. In this example, we use the Fisher’s exact test to find out.
[16]:
cc.tl.rank_peak_groups(adata_cc, 'Index', method = 'fisher_exact', key_added = 'fisher_exact')
100%|██████████| 2/2 [01:43<00:00, 51.87s/it]
Plot the results for differential peak analysis.
cc.tl.rank_peak_groups(adata_cc, 'Index', method = 'fisher_exact', key_added = 'fisher_exact',
rankby = 'logfoldchanges')
cc.pl.rank_peak_groups(adata_cc, key = 'fisher_exact',rankby = 'logfoldchanges')
[17]:
cc.pl.rank_peak_groups(adata_cc, key = 'fisher_exact', rankby = 'pvalues')
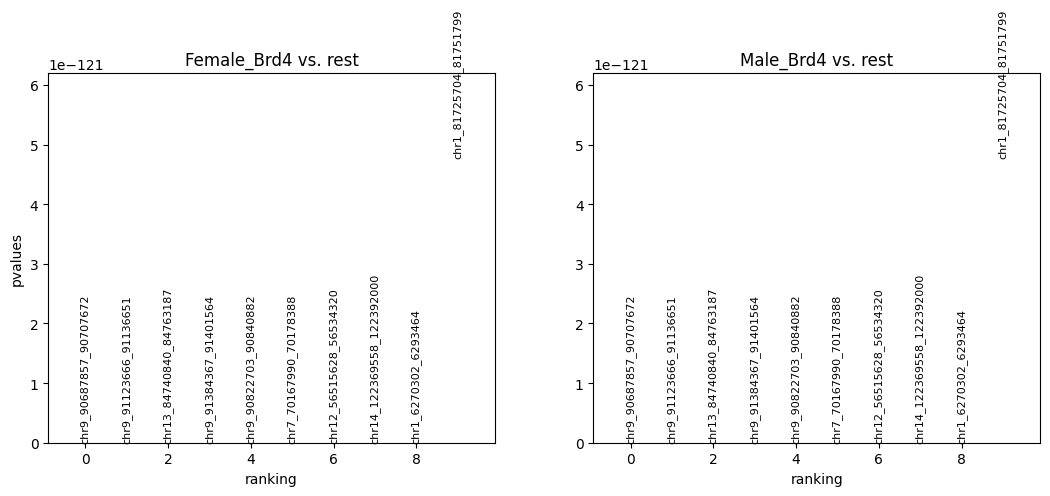
We can use Pycallingcards to look at the insertion densities at peaks that are differentially bound. In the plot below, we first plot female peaks as blue points and male as grey points, and then we plot male peaks as red and female peaks as grey. From the plots below we can see there is much more Brd4 binding at this locus in females than in males.
[18]:
cc.pl.draw_area("chr9", 91366534, 91425373, 50000, peak_data, Brd4, "mm10", adata = adata_cc, font_size=2,
plotsize = [1,1,4], name = "Female_Brd4",key = "Index",insertionkey = "group",
name_insertion1 = 'Female Insertions', name_density1 = 'Female Insertion Density',
name_insertion2 = 'Total Insertions', name_density2 = 'Total Insertion Density',
bins = 400, figsize = (30,12), peak_line = 6, title = "Female")
cc.pl.draw_area("chr9", 91366534, 91425373, 50000, peak_data, Brd4, "mm10", adata = adata_cc, font_size=2,
plotsize = [1,1,4], name = "Male_Brd4",key = "Index", insertionkey = "group",
name_insertion1 = 'Male Insertions', name_density1 = 'Male Insertion Density',
name_insertion2 = 'Total Insertions', name_density2 = 'Total Insertion Density',
bins = 400, figsize = (30,12), peak_line = 6, color = "blue", title = "Male")
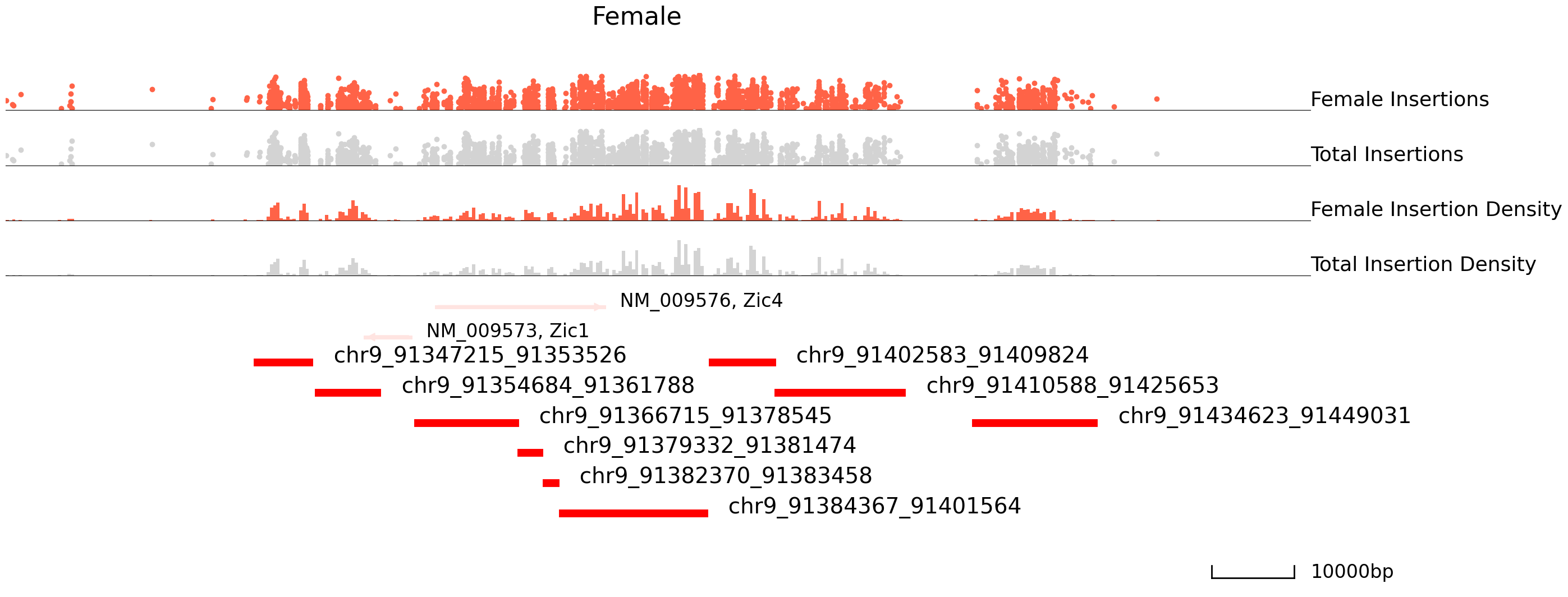
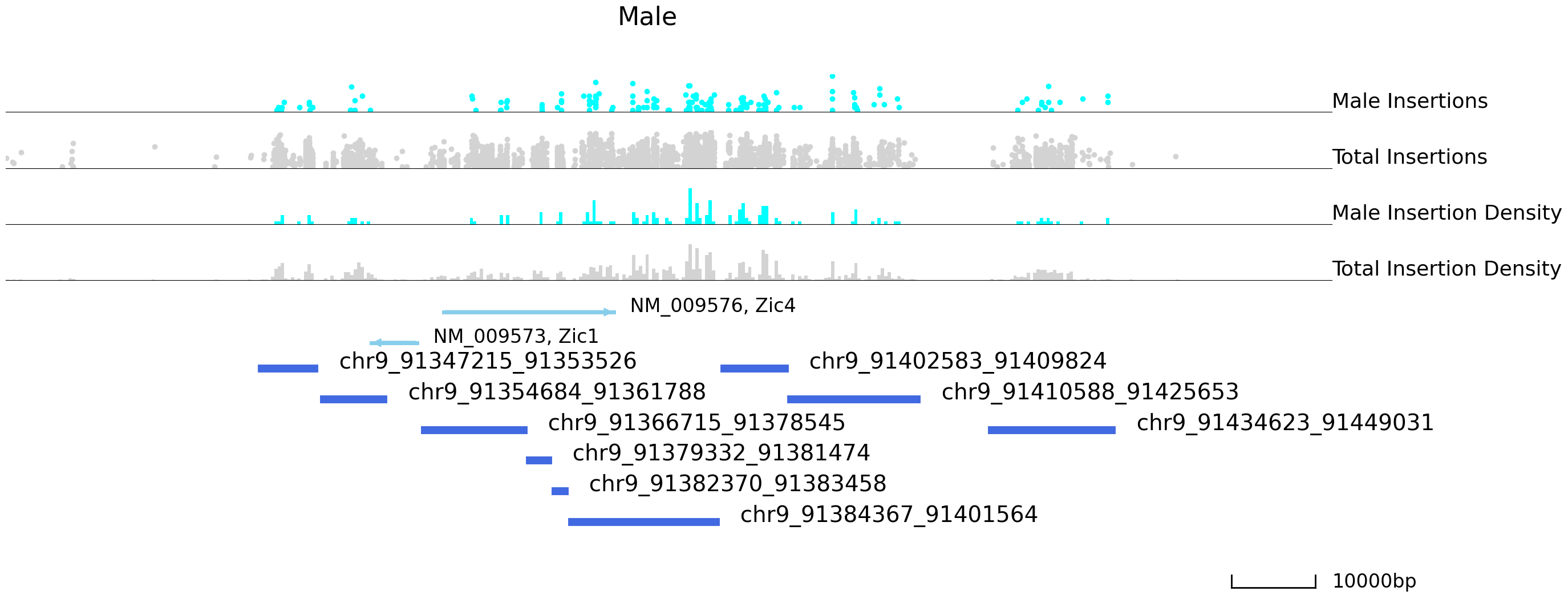
[19]:
cc.pl.draw_area("chr12", 56516453, 56538107, 50000, peak_data, Brd4, "mm10", adata = adata_cc, font_size=2,
plotsize = [1,1,3], name = "Female_Brd4", key = "Index",insertionkey = "group", bins = 200,
name_insertion1 = 'Female Insertions', name_density1 = 'Female Insertion Density',
name_insertion2 = 'Total Insertions', name_density2 = 'Total Insertion Density',
figsize = (30,8), peak_line = 2, title = "Female")
cc.pl.draw_area("chr12", 56516453, 56538107, 50000, peak_data, Brd4, "mm10", adata = adata_cc, font_size=2,
plotsize = [1,1,3], name = "Male_Brd4", key = "Index", insertionkey = "group", bins = 200,
name_insertion1 = 'Male Insertions', name_density1 = 'Male Insertion Density',
name_insertion2 = 'Total Insertions', name_density2 = 'Total Insertion Density',
figsize = (30,8), peak_line = 2, color = "blue", title = "Male")
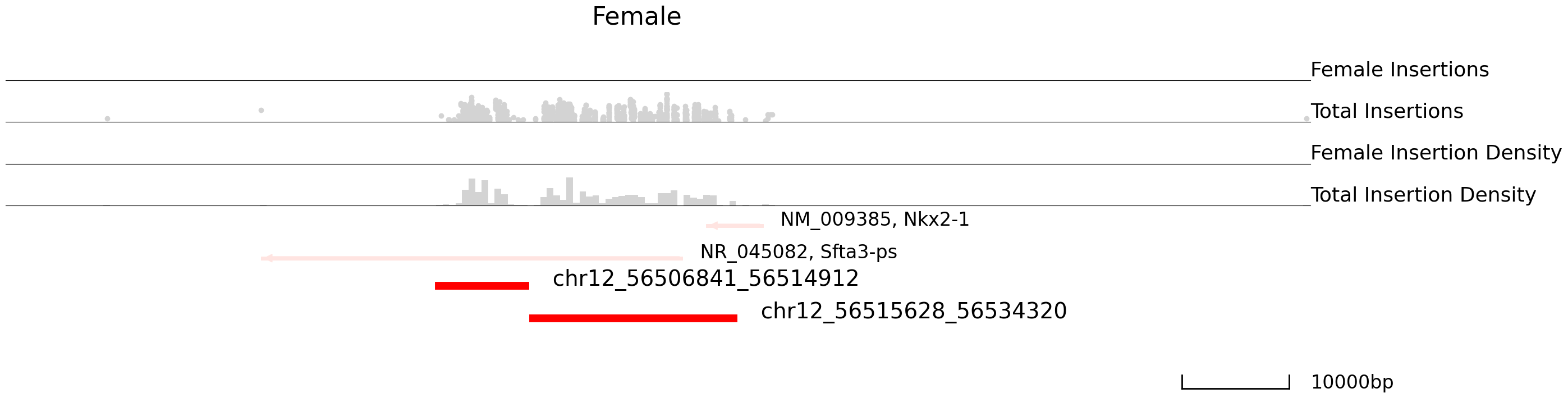
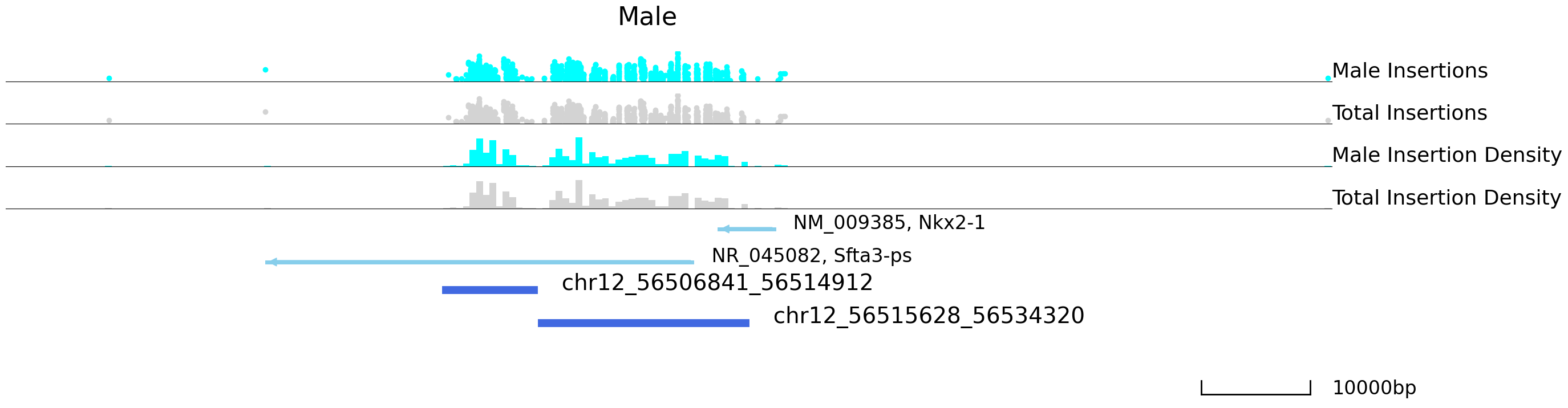
We can now plot a volcano plot to visualize the distribution of differentially bound peaks. labelright and labellest are the location points of the labels.
[20]:
cc.pl.volcano_plot(adata_cc, pvalue_name = 'pvalues_adj', pvalue_cutoff = 0.01, lfc_cutoff = 4,
figsize = (5,4), labelright = (3,250), labelleft = (-9,250))
/home/juanru/Desktop/pycallingcards/pycallingcards/pycallingcards/plotting/_volcano.py:70: RuntimeWarning: divide by zero encountered in log10
pva = -np.log10(np.array(adata_cc.uns[name][pvalue_name].tolist())[:, 1])
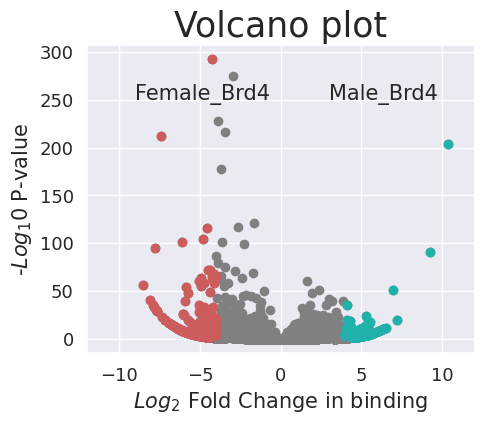
We can now use HOMER to call motifs for both female and male bound peaks separately to find out potential motif binding difference.
[31]:
cc.tl.call_motif(peaks_frame=cc.tl.rank_peak_groups_df(adata_cc, key = 'fisher_exact', pval_cutoff = 0.01, logfc_min = 3, group = ['Female_Brd4'])["names"].str.split("_", expand=True),
reference ="mm10",save_homer = "Homer/GBM_female",
homer_path = "/home/juanru/miniconda3/bin/", num_cores=12)
cc.tl.call_motif(peaks_frame=cc.tl.rank_peak_groups_df(adata_cc, key = 'fisher_exact', pval_cutoff = 0.01, logfc_min = 3, group = ['Male_Brd4'])["names"].str.split("_", expand=True),
reference ="mm10",save_homer = "Homer/GBM_male",
homer_path = "/home/juanru/miniconda3/bin/", num_cores=12)
There is no save_name, it will save to temp_Homer_trial.bed and then delete.
Position file = temp_Homer_trial.bed
Genome = mm10
Output Directory = Homer/GBM_female
Fragment size set to 1000
Using 12 CPUs
Will not run homer for de novo motifs
Found mset for "mouse", will check against vertebrates motifs
Peak/BED file conversion summary:
BED/Header formatted lines: 1009
peakfile formatted lines: 0
Peak File Statistics:
Total Peaks: 1009
Redundant Peak IDs: 0
Peaks lacking information: 0 (need at least 5 columns per peak)
Peaks with misformatted coordinates: 0 (should be integer)
Peaks with misformatted strand: 0 (should be either +/- or 0/1)
Peak file looks good!
Background files for 1000 bp fragments found.
Custom genome sequence directory: /home/juanru/miniconda3/share/homer/.//data/genomes/mm10//
Extracting sequences from directory: /home/juanru/miniconda3/share/homer/.//data/genomes/mm10//
Extracting 96 sequences from chr1
Extracting 93 sequences from chr2
Extracting 62 sequences from chr3
Extracting 39 sequences from chr4
Extracting 67 sequences from chr5
Extracting 66 sequences from chr6
Extracting 62 sequences from chr7
Extracting 45 sequences from chr8
Extracting 59 sequences from chr9
Extracting 50 sequences from chr10
Extracting 41 sequences from chr11
Extracting 41 sequences from chr12
Extracting 46 sequences from chr13
Extracting 25 sequences from chr14
Extracting 26 sequences from chr15
Extracting 40 sequences from chr16
Extracting 40 sequences from chr17
Extracting 32 sequences from chr18
Extracting 32 sequences from chr19
Extracting 47 sequences from chrX
Not removing redundant sequences
Sequences processed:
Auto detected maximum sequence length of 1001 bp
1009 total
Frequency Bins: 0.2 0.25 0.3 0.35 0.4 0.45 0.5 0.6 0.7 0.8
Freq Bin Count
0.3 2 14
0.35 3 101
0.4 4 317
0.45 5 309
0.5 6 201
0.6 7 66
0.7 8 1
Total sequences set to 50000
Choosing background that matches in CpG/GC content...
Bin # Targets # Background Background Weight
2 14 680 1.000
3 101 4904 1.000
4 317 15392 1.000
5 309 15003 1.000
6 201 9759 1.000
7 66 3205 1.000
8 1 49 0.991
Assembling sequence file...
Normalizing lower order oligos using homer2
Reading input files...
50001 total sequences read
Autonormalization: 1-mers (4 total)
A 29.26% 29.21% 1.001
C 20.74% 20.79% 0.998
G 20.74% 20.79% 0.998
T 29.26% 29.21% 1.001
Autonormalization: 2-mers (16 total)
AA 9.53% 9.22% 1.034
CA 7.34% 7.44% 0.987
GA 6.15% 6.15% 1.001
TA 6.23% 6.41% 0.972
AC 5.27% 5.38% 0.980
CC 5.08% 5.17% 0.983
GC 4.24% 4.08% 1.037
TC 6.15% 6.15% 1.001
AG 7.36% 7.34% 1.003
CG 0.96% 0.84% 1.148
GG 5.08% 5.17% 0.983
TG 7.34% 7.44% 0.987
AT 7.09% 7.28% 0.974
CT 7.36% 7.34% 1.003
GT 5.27% 5.38% 0.980
TT 9.53% 9.22% 1.034
Autonormalization: 3-mers (64 total)
Normalization weights can be found in file: Homer/GBM_female/seq.autonorm.tsv
Converging on autonormalization solution:
...............................................................................
Final normalization: Autonormalization: 1-mers (4 total)
A 29.26% 29.24% 1.001
C 20.74% 20.76% 0.999
G 20.74% 20.76% 0.999
T 29.26% 29.24% 1.001
Autonormalization: 2-mers (16 total)
AA 9.53% 9.41% 1.013
CA 7.34% 7.35% 0.998
GA 6.15% 6.14% 1.001
TA 6.23% 6.33% 0.985
AC 5.27% 5.32% 0.991
CC 5.08% 5.14% 0.989
GC 4.24% 4.16% 1.018
TC 6.15% 6.14% 1.001
AG 7.36% 7.34% 1.002
CG 0.96% 0.93% 1.033
GG 5.08% 5.14% 0.989
TG 7.34% 7.35% 0.998
AT 7.09% 7.16% 0.990
CT 7.36% 7.34% 1.002
GT 5.27% 5.32% 0.991
TT 9.53% 9.41% 1.013
Autonormalization: 3-mers (64 total)
Finished preparing sequence/group files
----------------------------------------------------------
Known motif enrichment
Reading input files...
50001 total sequences read
440 motifs loaded
Cache length = 11180
Using binomial scoring
Checking enrichment of 440 motif(s)
|0% 50% 100%|
=================================================================================
Preparing HTML output with sequence logos...
1 of 440 (1e-18) Chop(bZIP)/MEF-Chop-ChIP-Seq(GSE35681)/Homer
2 of 440 (1e-17) Atf4(bZIP)/MEF-Atf4-ChIP-Seq(GSE35681)/Homer
3 of 440 (1e-17) ISRE(IRF)/ThioMac-LPS-Expression(GSE23622)/Homer
4 of 440 (1e-15) Atf3(bZIP)/GBM-ATF3-ChIP-Seq(GSE33912)/Homer
5 of 440 (1e-15) Fos(bZIP)/TSC-Fos-ChIP-Seq(GSE110950)/Homer
6 of 440 (1e-15) AP-1(bZIP)/ThioMac-PU.1-ChIP-Seq(GSE21512)/Homer
7 of 440 (1e-15) JunB(bZIP)/DendriticCells-Junb-ChIP-Seq(GSE36099)/Homer
8 of 440 (1e-14) Fra1(bZIP)/BT549-Fra1-ChIP-Seq(GSE46166)/Homer
9 of 440 (1e-13) BATF(bZIP)/Th17-BATF-ChIP-Seq(GSE39756)/Homer
10 of 440 (1e-13) IRF2(IRF)/Erythroblas-IRF2-ChIP-Seq(GSE36985)/Homer
11 of 440 (1e-12) IRF3(IRF)/BMDM-Irf3-ChIP-Seq(GSE67343)/Homer
12 of 440 (1e-12) IRF8(IRF)/BMDM-IRF8-ChIP-Seq(GSE77884)/Homer
13 of 440 (1e-11) Fra2(bZIP)/Striatum-Fra2-ChIP-Seq(GSE43429)/Homer
14 of 440 (1e-11) Fosl2(bZIP)/3T3L1-Fosl2-ChIP-Seq(GSE56872)/Homer
15 of 440 (1e-10) Jun-AP1(bZIP)/K562-cJun-ChIP-Seq(GSE31477)/Homer
16 of 440 (1e-9) IRF1(IRF)/PBMC-IRF1-ChIP-Seq(GSE43036)/Homer
17 of 440 (1e-7) CEBP:AP1(bZIP)/ThioMac-CEBPb-ChIP-Seq(GSE21512)/Homer
18 of 440 (1e-7) Rbpj1(?)/Panc1-Rbpj1-ChIP-Seq(GSE47459)/Homer
19 of 440 (1e-7) TEAD1(TEAD)/HepG2-TEAD1-ChIP-Seq(Encode)/Homer
20 of 440 (1e-6) PU.1:IRF8(ETS:IRF)/pDC-Irf8-ChIP-Seq(GSE66899)/Homer
21 of 440 (1e-6) GABPA(ETS)/Jurkat-GABPa-ChIP-Seq(GSE17954)/Homer
22 of 440 (1e-6) Zic3(Zf)/mES-Zic3-ChIP-Seq(GSE37889)/Homer
23 of 440 (1e-5) Unknown-ESC-element(?)/mES-Nanog-ChIP-Seq(GSE11724)/Homer
24 of 440 (1e-4) ETS1(ETS)/Jurkat-ETS1-ChIP-Seq(GSE17954)/Homer
25 of 440 (1e-4) NF1-halfsite(CTF)/LNCaP-NF1-ChIP-Seq(Unpublished)/Homer
26 of 440 (1e-4) Mef2c(MADS)/GM12878-Mef2c-ChIP-Seq(GSE32465)/Homer
27 of 440 (1e-4) TEAD(TEA)/Fibroblast-PU.1-ChIP-Seq(Unpublished)/Homer
28 of 440 (1e-4) Isl1(Homeobox)/Neuron-Isl1-ChIP-Seq(GSE31456)/Homer
29 of 440 (1e-4) STAT5(Stat)/mCD4+-Stat5-ChIP-Seq(GSE12346)/Homer
30 of 440 (1e-4) TEAD3(TEA)/HepG2-TEAD3-ChIP-Seq(Encode)/Homer
31 of 440 (1e-4) CEBP:CEBP(bZIP)/MEF-Chop-ChIP-Seq(GSE35681)/Homer
32 of 440 (1e-4) TEAD4(TEA)/Tropoblast-Tead4-ChIP-Seq(GSE37350)/Homer
33 of 440 (1e-4) Pitx1:Ebox(Homeobox,bHLH)/Hindlimb-Pitx1-ChIP-Seq(GSE41591)/Homer
34 of 440 (1e-4) PAX6(Paired,Homeobox)/Forebrain-Pax6-ChIP-Seq(GSE66961)/Homer
35 of 440 (1e-4) Smad4(MAD)/ESC-SMAD4-ChIP-Seq(GSE29422)/Homer
36 of 440 (1e-4) Dlx3(Homeobox)/Kerainocytes-Dlx3-ChIP-Seq(GSE89884)/Homer
37 of 440 (1e-4) Fli1(ETS)/CD8-FLI-ChIP-Seq(GSE20898)/Homer
38 of 440 (1e-4) CREB5(bZIP)/LNCaP-CREB5.V5-ChIP-Seq(GSE137775)/Homer
39 of 440 (1e-3) EWS:FLI1-fusion(ETS)/SK_N_MC-EWS:FLI1-ChIP-Seq(SRA014231)/Homer
40 of 440 (1e-3) Bach2(bZIP)/OCILy7-Bach2-ChIP-Seq(GSE44420)/Homer
41 of 440 (1e-3) STAT4(Stat)/CD4-Stat4-ChIP-Seq(GSE22104)/Homer
42 of 440 (1e-3) IRF4(IRF)/GM12878-IRF4-ChIP-Seq(GSE32465)/Homer
43 of 440 (1e-3) Etv2(ETS)/ES-ER71-ChIP-Seq(GSE59402)/Homer
44 of 440 (1e-3) HLF(bZIP)/HSC-HLF.Flag-ChIP-Seq(GSE69817)/Homer
45 of 440 (1e-3) c-Jun-CRE(bZIP)/K562-cJun-ChIP-Seq(GSE31477)/Homer
46 of 440 (1e-3) AMYB(HTH)/Testes-AMYB-ChIP-Seq(GSE44588)/Homer
47 of 440 (1e-3) EWS:ERG-fusion(ETS)/CADO_ES1-EWS:ERG-ChIP-Seq(SRA014231)/Homer
48 of 440 (1e-3) TEAD2(TEA)/Py2T-Tead2-ChIP-Seq(GSE55709)/Homer
49 of 440 (1e-3) NFIL3(bZIP)/HepG2-NFIL3-ChIP-Seq(Encode)/Homer
50 of 440 (1e-3) ETV1(ETS)/GIST48-ETV1-ChIP-Seq(GSE22441)/Homer
51 of 440 (1e-3) Zic(Zf)/Cerebellum-ZIC1.2-ChIP-Seq(GSE60731)/Homer
52 of 440 (1e-3) Mef2a(MADS)/HL1-Mef2a.biotin-ChIP-Seq(GSE21529)/Homer
53 of 440 (1e-3) Elf4(ETS)/BMDM-Elf4-ChIP-Seq(GSE88699)/Homer
54 of 440 (1e-3) CArG(MADS)/PUER-Srf-ChIP-Seq(Sullivan_et_al.)/Homer
55 of 440 (1e-3) Elk1(ETS)/Hela-Elk1-ChIP-Seq(GSE31477)/Homer
56 of 440 (1e-2) MafA(bZIP)/Islet-MafA-ChIP-Seq(GSE30298)/Homer
57 of 440 (1e-2) MYB(HTH)/ERMYB-Myb-ChIPSeq(GSE22095)/Homer
58 of 440 (1e-2) PU.1-IRF(ETS:IRF)/Bcell-PU.1-ChIP-Seq(GSE21512)/Homer
59 of 440 (1e-2) EBF1(EBF)/Near-E2A-ChIP-Seq(GSE21512)/Homer
60 of 440 (1e-2) DLX5(Homeobox)/BasalGanglia-Dlx5-ChIP-seq(GSE124936)/Homer
61 of 440 (1e-2) Zic2(Zf)/ESC-Zic2-ChIP-Seq(SRP197560)/Homer
62 of 440 (1e-2) DLX1(Homeobox)/BasalGanglia-Dlx1-ChIP-seq(GSE124936)/Homer
63 of 440 (1e-2) Six2(Homeobox)/NephronProgenitor-Six2-ChIP-Seq(GSE39837)/Homer
64 of 440 (1e-2) STAT6(Stat)/Macrophage-Stat6-ChIP-Seq(GSE38377)/Homer
65 of 440 (1e-2) NFAT:AP1(RHD,bZIP)/Jurkat-NFATC1-ChIP-Seq(Jolma_et_al.)/Homer
66 of 440 (1e-2) Fox:Ebox(Forkhead,bHLH)/Panc1-Foxa2-ChIP-Seq(GSE47459)/Homer
67 of 440 (1e-2) Stat3(Stat)/mES-Stat3-ChIP-Seq(GSE11431)/Homer
68 of 440 (1e-2) NFkB-p65-Rel(RHD)/ThioMac-LPS-Expression(GSE23622)/Homer
69 of 440 (1e-2) EHF(ETS)/LoVo-EHF-ChIP-Seq(GSE49402)/Homer
70 of 440 (1e-2) Hoxd11(Homeobox)/ChickenMSG-Hoxd11.Flag-ChIP-Seq(GSE86088)/Homer
71 of 440 (1e-2) IRF:BATF(IRF:bZIP)/pDC-Irf8-ChIP-Seq(GSE66899)/Homer
72 of 440 (1e-2) ETV4(ETS)/HepG2-ETV4-ChIP-Seq(ENCODE)/Homer
73 of 440 (1e-2) Smad2(MAD)/ES-SMAD2-ChIP-Seq(GSE29422)/Homer
74 of 440 (1e-2) STAT6(Stat)/CD4-Stat6-ChIP-Seq(GSE22104)/Homer
75 of 440 (1e-2) Prop1(Homeobox)/GHFT1-PROP1.biotin-ChIP-Seq(GSE77302)/Homer
76 of 440 (1e-2) Brn2(POU,Homeobox)/NPC-Brn2-ChIP-Seq(GSE35496)/Homer
77 of 440 (1e-2) NFkB-p65(RHD)/GM12787-p65-ChIP-Seq(GSE19485)/Homer
78 of 440 (1e-2) ETS:E-box(ETS,bHLH)/HPC7-Scl-ChIP-Seq(GSE22178)/Homer
79 of 440 (1e-2) PU.1(ETS)/ThioMac-PU.1-ChIP-Seq(GSE21512)/Homer
80 of 440 (1e-2) Nkx6.1(Homeobox)/Islet-Nkx6.1-ChIP-Seq(GSE40975)/Homer
81 of 440 (1e-2) Mef2d(MADS)/Retina-Mef2d-ChIP-Seq(GSE61391)/Homer
Skipping...
Job finished - if results look good, please send beer to ..
Cleaning up tmp files...
Position file = temp_Homer_trial.bed
Genome = mm10
Output Directory = Homer/GBM_male
Fragment size set to 1000
Using 12 CPUs
Will not run homer for de novo motifs
Found mset for "mouse", will check against vertebrates motifs
Peak/BED file conversion summary:
BED/Header formatted lines: 585
peakfile formatted lines: 0
Peak File Statistics:
Total Peaks: 585
Redundant Peak IDs: 0
Peaks lacking information: 0 (need at least 5 columns per peak)
Peaks with misformatted coordinates: 0 (should be integer)
Peaks with misformatted strand: 0 (should be either +/- or 0/1)
Peak file looks good!
Finished!
There is no save_name, it will save to temp_Homer_trial.bed and then delete.
Background files for 1000 bp fragments found.
Custom genome sequence directory: /home/juanru/miniconda3/share/homer/.//data/genomes/mm10//
Extracting sequences from directory: /home/juanru/miniconda3/share/homer/.//data/genomes/mm10//
Extracting 36 sequences from chr1
Extracting 40 sequences from chr2
Extracting 33 sequences from chr3
Extracting 20 sequences from chr4
Extracting 28 sequences from chr5
Extracting 35 sequences from chr6
Extracting 18 sequences from chr7
Extracting 25 sequences from chr8
Extracting 21 sequences from chr9
Extracting 52 sequences from chr10
Extracting 39 sequences from chr11
Extracting 26 sequences from chr12
Extracting 23 sequences from chr13
Extracting 36 sequences from chr14
Extracting 43 sequences from chr15
Extracting 18 sequences from chr16
Extracting 17 sequences from chr17
Extracting 28 sequences from chr18
Extracting 22 sequences from chr19
Extracting 21 sequences from chrX
Extracting 4 sequences from chrY
Not removing redundant sequences
Sequences processed:
Auto detected maximum sequence length of 1001 bp
585 total
Frequency Bins: 0.2 0.25 0.3 0.35 0.4 0.45 0.5 0.6 0.7 0.8
Freq Bin Count
0.3 2 2
0.35 3 41
0.4 4 134
0.45 5 215
0.5 6 133
0.6 7 56
0.7 8 4
Total sequences set to 50000
Choosing background that matches in CpG/GC content...
Bin # Targets # Background Background Weight
2 2 169 1.000
3 41 3463 1.000
4 134 11319 1.000
5 215 18161 1.000
6 133 11235 1.000
7 56 4730 1.000
8 4 338 1.000
Assembling sequence file...
Normalizing lower order oligos using homer2
Reading input files...
50000 total sequences read
Autonormalization: 1-mers (4 total)
A 28.57% 28.51% 1.002
C 21.43% 21.49% 0.997
G 21.43% 21.49% 0.997
T 28.57% 28.51% 1.002
Autonormalization: 2-mers (16 total)
AA 9.11% 8.75% 1.041
CA 7.48% 7.54% 0.993
GA 6.12% 6.21% 0.985
TA 5.87% 6.02% 0.974
AC 5.39% 5.41% 0.995
CC 5.41% 5.51% 0.982
GC 4.51% 4.35% 1.035
TC 6.12% 6.21% 0.985
AG 7.40% 7.49% 0.988
CG 1.13% 0.95% 1.196
GG 5.41% 5.51% 0.982
TG 7.48% 7.54% 0.993
AT 6.67% 6.86% 0.973
CT 7.40% 7.49% 0.988
GT 5.39% 5.41% 0.995
TT 9.11% 8.75% 1.041
Autonormalization: 3-mers (64 total)
Normalization weights can be found in file: Homer/GBM_male/seq.autonorm.tsv
Converging on autonormalization solution:
...............................................................................
Final normalization: Autonormalization: 1-mers (4 total)
A 28.57% 28.55% 1.001
C 21.43% 21.45% 0.999
G 21.43% 21.45% 0.999
T 28.57% 28.55% 1.001
Autonormalization: 2-mers (16 total)
AA 9.11% 8.97% 1.016
CA 7.48% 7.47% 1.001
GA 6.12% 6.15% 0.995
TA 5.87% 5.97% 0.983
AC 5.39% 5.40% 0.998
CC 5.41% 5.46% 0.992
GC 4.51% 4.44% 1.014
TC 6.12% 6.15% 0.995
AG 7.40% 7.42% 0.997
CG 1.13% 1.10% 1.033
GG 5.41% 5.46% 0.992
TG 7.48% 7.47% 1.001
AT 6.67% 6.77% 0.986
CT 7.40% 7.42% 0.997
GT 5.39% 5.40% 0.998
TT 9.11% 8.97% 1.016
Autonormalization: 3-mers (64 total)
Finished preparing sequence/group files
----------------------------------------------------------
Known motif enrichment
Reading input files...
50000 total sequences read
440 motifs loaded
Cache length = 11180
Using binomial scoring
Checking enrichment of 440 motif(s)
|0% 50% 100%|
=================================================================================
Finished!
Preparing HTML output with sequence logos...
1 of 440 (1e-42) Fosl2(bZIP)/3T3L1-Fosl2-ChIP-Seq(GSE56872)/Homer
2 of 440 (1e-41) Jun-AP1(bZIP)/K562-cJun-ChIP-Seq(GSE31477)/Homer
3 of 440 (1e-39) Fos(bZIP)/TSC-Fos-ChIP-Seq(GSE110950)/Homer
4 of 440 (1e-38) JunB(bZIP)/DendriticCells-Junb-ChIP-Seq(GSE36099)/Homer
5 of 440 (1e-37) Fra1(bZIP)/BT549-Fra1-ChIP-Seq(GSE46166)/Homer
6 of 440 (1e-35) Fra2(bZIP)/Striatum-Fra2-ChIP-Seq(GSE43429)/Homer
7 of 440 (1e-35) BATF(bZIP)/Th17-BATF-ChIP-Seq(GSE39756)/Homer
8 of 440 (1e-34) Atf3(bZIP)/GBM-ATF3-ChIP-Seq(GSE33912)/Homer
9 of 440 (1e-34) AP-1(bZIP)/ThioMac-PU.1-ChIP-Seq(GSE21512)/Homer
10 of 440 (1e-22) Bach2(bZIP)/OCILy7-Bach2-ChIP-Seq(GSE44420)/Homer
11 of 440 (1e-10) Nrf2(bZIP)/Lymphoblast-Nrf2-ChIP-Seq(GSE37589)/Homer
12 of 440 (1e-9) NF-E2(bZIP)/K562-NFE2-ChIP-Seq(GSE31477)/Homer
13 of 440 (1e-7) Bach1(bZIP)/K562-Bach1-ChIP-Seq(GSE31477)/Homer
14 of 440 (1e-7) NFE2L2(bZIP)/HepG2-NFE2L2-ChIP-Seq(Encode)/Homer
15 of 440 (1e-6) MafK(bZIP)/C2C12-MafK-ChIP-Seq(GSE36030)/Homer
16 of 440 (1e-5) Ets1-distal(ETS)/CD4+-PolII-ChIP-Seq(Barski_et_al.)/Homer
17 of 440 (1e-4) Isl1(Homeobox)/Neuron-Isl1-ChIP-Seq(GSE31456)/Homer
18 of 440 (1e-4) Sox3(HMG)/NPC-Sox3-ChIP-Seq(GSE33059)/Homer
19 of 440 (1e-3) Bapx1(Homeobox)/VertebralCol-Bapx1-ChIP-Seq(GSE36672)/Homer
20 of 440 (1e-3) Fli1(ETS)/CD8-FLI-ChIP-Seq(GSE20898)/Homer
21 of 440 (1e-3) MafA(bZIP)/Islet-MafA-ChIP-Seq(GSE30298)/Homer
22 of 440 (1e-3) Fox:Ebox(Forkhead,bHLH)/Panc1-Foxa2-ChIP-Seq(GSE47459)/Homer
23 of 440 (1e-3) Nkx2.2(Homeobox)/NPC-Nkx2.2-ChIP-Seq(GSE61673)/Homer
24 of 440 (1e-3) Sox10(HMG)/SciaticNerve-Sox3-ChIP-Seq(GSE35132)/Homer
25 of 440 (1e-3) LHX9(Homeobox)/Hct116-LHX9.V5-ChIP-Seq(GSE116822)/Homer
26 of 440 (1e-3) TATA-Box(TBP)/Promoter/Homer
27 of 440 (1e-3) KLF14(Zf)/HEK293-KLF14.GFP-ChIP-Seq(GSE58341)/Homer
28 of 440 (1e-2) ZNF669(Zf)/HEK293-ZNF669.GFP-ChIP-Seq(GSE58341)/Homer
29 of 440 (1e-2) Lhx2(Homeobox)/HFSC-Lhx2-ChIP-Seq(GSE48068)/Homer
30 of 440 (1e-2) Sox4(HMG)/proB-Sox4-ChIP-Seq(GSE50066)/Homer
31 of 440 (1e-2) DLX5(Homeobox)/BasalGanglia-Dlx5-ChIP-seq(GSE124936)/Homer
32 of 440 (1e-2) RUNX-AML(Runt)/CD4+-PolII-ChIP-Seq(Barski_et_al.)/Homer
33 of 440 (1e-2) Sp5(Zf)/mES-Sp5.Flag-ChIP-Seq(GSE72989)/Homer
34 of 440 (1e-2) EWS:ERG-fusion(ETS)/CADO_ES1-EWS:ERG-ChIP-Seq(SRA014231)/Homer
35 of 440 (1e-2) RUNX1(Runt)/Jurkat-RUNX1-ChIP-Seq(GSE29180)/Homer
36 of 440 (1e-2) Etv2(ETS)/ES-ER71-ChIP-Seq(GSE59402)/Homer
37 of 440 (1e-2) ETV4(ETS)/HepG2-ETV4-ChIP-Seq(ENCODE)/Homer
38 of 440 (1e-2) Sox2(HMG)/mES-Sox2-ChIP-Seq(GSE11431)/Homer
39 of 440 (1e-2) ETS1(ETS)/Jurkat-ETS1-ChIP-Seq(GSE17954)/Homer
40 of 440 (1e-2) Brn1(POU,Homeobox)/NPC-Brn1-ChIP-Seq(GSE35496)/Homer
41 of 440 (1e-2) CHR(?)/Hela-CellCycle-Expression/Homer
42 of 440 (1e-2) Dlx3(Homeobox)/Kerainocytes-Dlx3-ChIP-Seq(GSE89884)/Homer
43 of 440 (1e-2) EWS:FLI1-fusion(ETS)/SK_N_MC-EWS:FLI1-ChIP-Seq(SRA014231)/Homer
44 of 440 (1e-2) KLF3(Zf)/MEF-Klf3-ChIP-Seq(GSE44748)/Homer
45 of 440 (1e-2) STAT5(Stat)/mCD4+-Stat5-ChIP-Seq(GSE12346)/Homer
46 of 440 (1e-2) Elk4(ETS)/Hela-Elk4-ChIP-Seq(GSE31477)/Homer
47 of 440 (1e-2) Tbx20(T-box)/Heart-Tbx20-ChIP-Seq(GSE29636)/Homer
48 of 440 (1e-2) Rfx6(HTH)/Min6b1-Rfx6.HA-ChIP-Seq(GSE62844)/Homer
49 of 440 (1e-2) ETS:RUNX(ETS,Runt)/Jurkat-RUNX1-ChIP-Seq(GSE17954)/Homer
50 of 440 (1e-2) AMYB(HTH)/Testes-AMYB-ChIP-Seq(GSE44588)/Homer
51 of 440 (1e-2) Lhx1(Homeobox)/EmbryoCarcinoma-Lhx1-ChIP-Seq(GSE70957)/Homer
52 of 440 (1e-2) Lhx3(Homeobox)/Neuron-Lhx3-ChIP-Seq(GSE31456)/Homer
53 of 440 (1e-2) DLX2(Homeobox)/BasalGanglia-Dlx2-ChIP-seq(GSE124936)/Homer
54 of 440 (1e-2) Nkx6.1(Homeobox)/Islet-Nkx6.1-ChIP-Seq(GSE40975)/Homer
55 of 440 (1e-2) OCT4-SOX2-TCF-NANOG(POU,Homeobox,HMG)/mES-Oct4-ChIP-Seq(GSE11431)/Homer
56 of 440 (1e-2) Sox7(HMG)/ESC-Sox7-ChIP-Seq(GSE133899)/Homer
57 of 440 (1e-2) Sox6(HMG)/Myotubes-Sox6-ChIP-Seq(GSE32627)/Homer
58 of 440 (1e-2) DLX1(Homeobox)/BasalGanglia-Dlx1-ChIP-seq(GSE124936)/Homer
59 of 440 (1e-2) AP-2alpha(AP2)/Hela-AP2alpha-ChIP-Seq(GSE31477)/Homer
60 of 440 (1e-2) GABPA(ETS)/Jurkat-GABPa-ChIP-Seq(GSE17954)/Homer
61 of 440 (1e-2) Maz(Zf)/HepG2-Maz-ChIP-Seq(GSE31477)/Homer
Skipping...
Job finished - if results look good, please send beer to ..
Cleaning up tmp files...
Find motifs under male peaks but not in female peaks.
[22]:
cc.tl.compare_motif("Homer/GBM_male", "Homer/GBM_female")
[22]:
Motif Name | Consensus | P-value | Log P-value | q-value (Benjamini) | # of Target Sequences with Motif(of 585) | % of Target Sequences with Motif | # of Background Sequences with Motif(of 2133) | % of Background Sequences with Motif | |
---|---|---|---|---|---|---|---|---|---|
10 | Nrf2(bZIP)/Lymphoblast-Nrf2-ChIP-Seq(GSE37589)... | HTGCTGAGTCAT | 1.000000e-13 | -30.290 | 0.0000 | 39.0 | 6.67% | 33.5 | 1.57% |
11 | NF-E2(bZIP)/K562-NFE2-ChIP-Seq(GSE31477)/Homer | GATGACTCAGCA | 1.000000e-11 | -26.520 | 0.0000 | 41.0 | 7.01% | 41.6 | 1.95% |
12 | NFE2L2(bZIP)/HepG2-NFE2L2-ChIP-Seq(Encode)/Homer | AWWWTGCTGAGTCAT | 1.000000e-11 | -25.480 | 0.0000 | 48.0 | 8.21% | 56.4 | 2.65% |
13 | Bach1(bZIP)/K562-Bach1-ChIP-Seq(GSE31477)/Homer | AWWNTGCTGAGTCAT | 1.000000e-09 | -21.840 | 0.0000 | 39.0 | 6.67% | 44.3 | 2.07% |
14 | MafK(bZIP)/C2C12-MafK-ChIP-Seq(GSE36030)/Homer | GCTGASTCAGCA | 1.000000e-09 | -20.870 | 0.0000 | 97.0 | 16.58% | 186.9 | 8.76% |
15 | Ets1-distal(ETS)/CD4+-PolII-ChIP-Seq(Barski_et... | MACAGGAAGT | 1.000000e-05 | -13.680 | 0.0000 | 116.0 | 19.83% | 273.6 | 12.82% |
17 | Sox3(HMG)/NPC-Sox3-ChIP-Seq(GSE33059)/Homer | CCWTTGTY | 1.000000e-04 | -9.625 | 0.0016 | 434.0 | 74.19% | 1425.7 | 66.82% |
18 | Nkx2.2(Homeobox)/NPC-Nkx2.2-ChIP-Seq(GSE61673)... | BTBRAGTGSN | 1.000000e-04 | -9.612 | 0.0016 | 419.0 | 71.62% | 1367.5 | 64.09% |
19 | Bapx1(Homeobox)/VertebralCol-Bapx1-ChIP-Seq(GS... | TTRAGTGSYK | 1.000000e-04 | -9.553 | 0.0016 | 467.0 | 79.83% | 1556.7 | 72.96% |
20 | RUNX1(Runt)/Jurkat-RUNX1-ChIP-Seq(GSE29180)/Homer | AAACCACARM | 1.000000e-03 | -8.988 | 0.0026 | 293.0 | 50.09% | 906.6 | 42.49% |
23 | ZNF669(Zf)/HEK293-ZNF669.GFP-ChIP-Seq(GSE58341... | GARTGGTCATCGCCC | 1.000000e-03 | -8.215 | 0.0050 | 24.0 | 4.10% | 39.7 | 1.86% |
27 | RUNX-AML(Runt)/CD4+-PolII-ChIP-Seq(Barski_et_a... | GCTGTGGTTW | 1.000000e-03 | -7.530 | 0.0086 | 212.0 | 36.24% | 637.6 | 29.89% |
29 | Sox10(HMG)/SciaticNerve-Sox3-ChIP-Seq(GSE35132... | CCWTTGTYYB | 1.000000e-03 | -7.342 | 0.0095 | 407.0 | 69.57% | 1347.5 | 63.15% |
30 | Sp1(Zf)/Promoter/Homer | GGCCCCGCCCCC | 1.000000e-03 | -7.220 | 0.0104 | 31.0 | 5.30% | 60.7 | 2.85% |
32 | E2F3(E2F)/MEF-E2F3-ChIP-Seq(GSE71376)/Homer | BTKGGCGGGAAA | 1.000000e-03 | -7.074 | 0.0113 | 77.0 | 13.16% | 195.8 | 9.18% |
37 | Sox7(HMG)/ESC-Sox7-ChIP-Seq(GSE133899)/Homer | VVRRAACAATGG | 1.000000e-02 | -6.569 | 0.0162 | 102.0 | 17.44% | 278.9 | 13.07% |
40 | Brn1(POU,Homeobox)/NPC-Brn1-ChIP-Seq(GSE35496)... | TATGCWAATBAV | 1.000000e-02 | -6.345 | 0.0188 | 134.0 | 22.91% | 385.4 | 18.06% |
42 | Sox4(HMG)/proB-Sox4-ChIP-Seq(GSE50066)/Homer | YCTTTGTTCC | 1.000000e-02 | -6.213 | 0.0205 | 255.0 | 43.59% | 804.0 | 37.68% |
43 | ZNF317(Zf)/HEK293-ZNF317.GFP-ChIP-Seq(GSE58341... | GTCWGCTGTYYCTCT | 1.000000e-02 | -6.112 | 0.0222 | 29.0 | 4.96% | 59.0 | 2.77% |
45 | OCT4-SOX2-TCF-NANOG(POU,Homeobox,HMG)/mES-Oct4... | ATTTGCATAACAATG | 1.000000e-02 | -5.636 | 0.0341 | 96.0 | 16.41% | 267.1 | 12.52% |
46 | Isl1(Homeobox)/Neuron-Isl1-ChIP-Seq(GSE31456)/... | CTAATKGV | 1.000000e-02 | -5.578 | 0.0354 | 467.0 | 79.83% | 1601.5 | 75.06% |
48 | Sox2(HMG)/mES-Sox2-ChIP-Seq(GSE11431)/Homer | BCCATTGTTC | 1.000000e-02 | -5.408 | 0.0402 | 272.0 | 46.50% | 876.6 | 41.09% |
49 | Egr1(Zf)/K562-Egr1-ChIP-Seq(GSE32465)/Homer | TGCGTGGGYG | 1.000000e-02 | -5.339 | 0.0422 | 137.0 | 23.42% | 406.2 | 19.04% |
50 | Tbx20(T-box)/Heart-Tbx20-ChIP-Seq(GSE29636)/Homer | GGTGYTGACAGS | 1.000000e-02 | -5.310 | 0.0426 | 67.0 | 11.45% | 178.0 | 8.34% |
51 | Nkx3.1(Homeobox)/LNCaP-Nkx3.1-ChIP-Seq(GSE2826... | AAGCACTTAA | 1.000000e-02 | -5.277 | 0.0432 | 479.0 | 81.88% | 1652.0 | 77.43% |
52 | E2F6(E2F)/Hela-E2F6-ChIP-Seq(GSE31477)/Homer | GGCGGGAARN | 1.000000e-02 | -5.256 | 0.0433 | 64.0 | 10.94% | 168.7 | 7.91% |
53 | TATA-Box(TBP)/Promoter/Homer | CCTTTTAWAGSC | 1.000000e-02 | -5.201 | 0.0449 | 382.0 | 65.30% | 1282.4 | 60.10% |
55 | Klf9(Zf)/GBM-Klf9-ChIP-Seq(GSE62211)/Homer | GCCACRCCCACY | 1.000000e-02 | -5.137 | 0.0462 | 85.0 | 14.53% | 236.3 | 11.08% |
Find motifs in female but not in male.
[23]:
cc.tl.compare_motif("Homer/GBM_female", "Homer/GBM_male")
[23]:
Motif Name | Consensus | P-value | Log P-value | q-value (Benjamini) | # of Target Sequences with Motif(of 1009) | % of Target Sequences with Motif | # of Background Sequences with Motif(of 2327) | % of Background Sequences with Motif | |
---|---|---|---|---|---|---|---|---|---|
0 | Atf4(bZIP)/MEF-Atf4-ChIP-Seq(GSE35681)/Homer | MTGATGCAAT | 1.000000e-14 | -33.810 | 0.0000 | 228.0 | 22.60% | 313.9 | 13.49% |
1 | Chop(bZIP)/MEF-Chop-ChIP-Seq(GSE35681)/Homer | ATTGCATCAT | 1.000000e-14 | -32.890 | 0.0000 | 197.0 | 19.52% | 259.5 | 11.15% |
2 | IRF8(IRF)/BMDM-IRF8-ChIP-Seq(GSE77884)/Homer | GRAASTGAAAST | 1.000000e-12 | -28.550 | 0.0000 | 255.0 | 25.27% | 381.5 | 16.40% |
5 | EBF1(EBF)/Near-E2A-ChIP-Seq(GSE21512)/Homer | GTCCCCWGGGGA | 1.000000e-11 | -27.250 | 0.0000 | 310.0 | 30.72% | 495.0 | 21.27% |
8 | Zic3(Zf)/mES-Zic3-ChIP-Seq(GSE37889)/Homer | GGCCYCCTGCTGDGH | 1.000000e-11 | -25.440 | 0.0000 | 189.0 | 18.73% | 266.5 | 11.45% |
... | ... | ... | ... | ... | ... | ... | ... | ... | ... |
122 | SPDEF(ETS)/VCaP-SPDEF-ChIP-Seq(SRA014231)/Homer | ASWTCCTGBT | 1.000000e-02 | -4.786 | 0.0299 | 420.0 | 41.63% | 882.4 | 37.92% |
123 | Srebp2(bHLH)/HepG2-Srebp2-ChIP-Seq(GSE31477)/H... | CGGTCACSCCAC | 1.000000e-02 | -4.760 | 0.0304 | 45.0 | 4.46% | 71.8 | 3.08% |
124 | ZNF519(Zf)/HEK293-ZNF519.GFP-ChIP-Seq(GSE58341... | GAGSCCGAGC | 1.000000e-02 | -4.720 | 0.0314 | 37.0 | 3.67% | 56.1 | 2.41% |
125 | AP-2alpha(AP2)/Hela-AP2alpha-ChIP-Seq(GSE31477... | ATGCCCTGAGGC | 1.000000e-02 | -4.695 | 0.0319 | 167.0 | 16.55% | 323.9 | 13.92% |
126 | Atf7(bZIP)/3T3L1-Atf7-ChIP-Seq(GSE56872)/Homer | NGRTGACGTCAY | 1.000000e-02 | -4.676 | 0.0323 | 192.0 | 19.03% | 377.5 | 16.22% |
98 rows × 9 columns
Next, we want to identify differentially bound peaks whose nearby genes are differentially expressed between males and females. To do so, we must first read in the bulk RNA-seq data. Note that this RNA-seq data is normalized by RPKM.
[24]:
rna = cc.datasets.mouse_brd4_data(data = "RNA")
rna
[24]:
sample.f6_dmso_3 | sample.f6_dmso_2 | sample.f6_dmso_1 | sample.m6_dmso_3 | sample.m6_dmso_2 | sample.m6_dmso_1 | |
---|---|---|---|---|---|---|
mt-Tf | 0.000000 | 0.639272 | 0.000000 | 1.156308 | 0.000000 | 0.687531 |
mt-Rnr1 | 127.907281 | 184.396768 | 172.544723 | 130.417054 | 192.566581 | 114.212201 |
mt-Tv | 0.000000 | 0.000000 | 1.761150 | 0.000000 | 0.510777 | 0.000000 |
mt-Rnr2 | 247.267306 | 527.855824 | 324.345998 | 675.031187 | 684.376279 | 418.345603 |
mt-Tl1 | 44.418710 | 117.660098 | 70.481211 | 167.217607 | 115.129081 | 127.789076 |
... | ... | ... | ... | ... | ... | ... |
Gm16897 | 0.281553 | 0.349020 | 0.463442 | 0.078913 | 0.070742 | 0.112610 |
A330023F24Rik | 0.118678 | 0.055749 | 0.058441 | 0.029411 | 0.018833 | 0.049965 |
Cd46 | 0.127930 | 0.095151 | 0.075997 | 0.061467 | 0.055103 | 0.087715 |
Cr1l | 9.993119 | 14.027801 | 13.717564 | 13.586101 | 13.240219 | 11.089902 |
Cr2 | 0.018832 | 0.056028 | 0.019578 | 0.012668 | 0.017034 | 0.015064 |
21430 rows × 6 columns
We now plot the heatmap for calling cards data and gene expression data.
The top lines are the relative Brd4 binding for each of the two groups. The bottom lines are the gene expressions of the top two nearest genes in the 3 RNA-seq replicates for males and females; each gene shown separately.
We can see a relationship between Brd4 binding and the expression of nearby genes. Genes that are strongly bound in females tend to be expressed in females and expressed at lower levels in males and vice versa. The effect is much more muted for the 2nd nearest gene to the peak.
[25]:
cc.pl.heatmap(adata_cc,rna, rnalabels = ["Female1", "Female2", "Female3","Male1", "Male2", "Male3"])
Please make sure that the samples in adata_cc and rna are in the same order.
/home/juanru/Desktop/pycallingcards/pycallingcards/pycallingcards/plotting/_heatmap_ccrna.py:193: RuntimeWarning: invalid value encountered in true_divide
data[groupnumber:secondnum, :]
/home/juanru/Desktop/pycallingcards/pycallingcards/pycallingcards/plotting/_heatmap_ccrna.py:195: RuntimeWarning: invalid value encountered in true_divide
data[secondnum:, :]
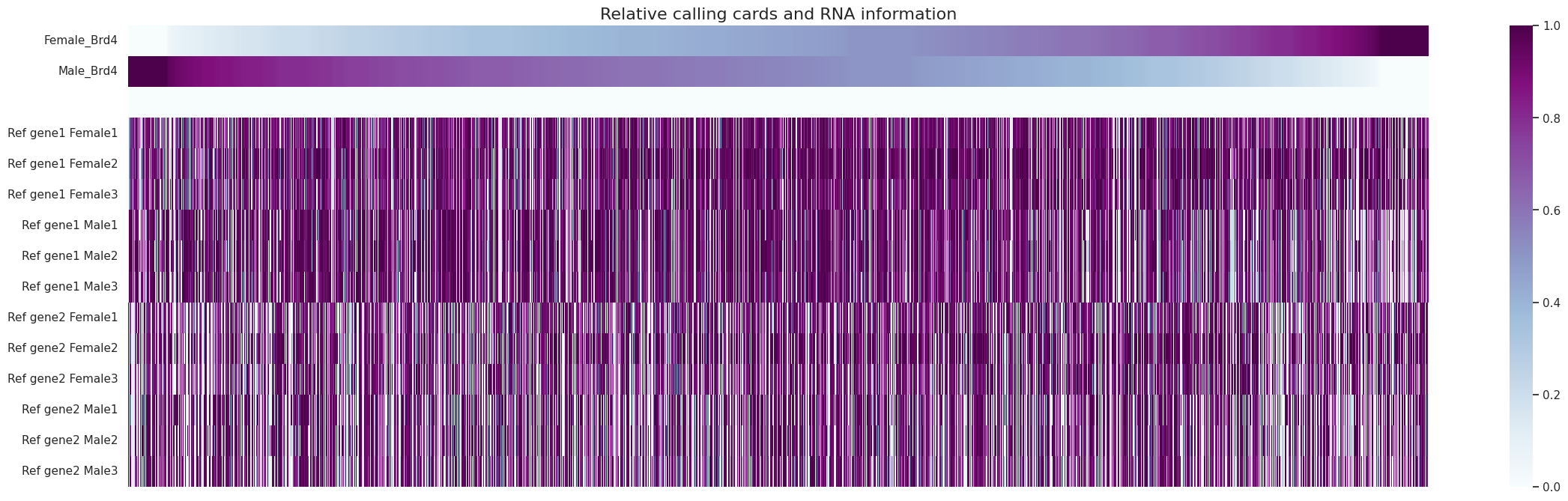
Find out the co-differential peak-gene pairs. Look into all the differential peaks and then see if the annotated genes are also significantly expressed. We can set the pvalue and score/log foldchange cutoff easily.
[26]:
result = cc.tl.pair_peak_gene_bulk(adata_cc, "https://github.com/The-Mitra-Lab/pycallingcards_data/releases/download/data/deseq_MF.csv",
name_cc = 'logfoldchanges', pvalue_cutoff_cc = 0.001, pvalue_cutoff_rna = 0.001,
lfc_cutoff_rna = 5, lfc_cutoff_cc = 5)
result
[26]:
Peak | logfoldchanges_peak | Pvalue_peak | Pvalue_adj_peak | Gene | Score_gene | Pvalue_gene | Pvalue_adj_gene | Distance_peak_to_gene | |
---|---|---|---|---|---|---|---|---|---|
0 | chr1_174659622_174662521 | 5.652435 | 1.530047e-08 | 4.586591e-07 | Grem2 | -10.401831 | 1.280025e-67 | 1.252748e-65 | 171264 |
1 | chr1_174917639_174921559 | 6.208699 | 2.711848e-12 | 1.562878e-10 | Grem2 | -10.401831 | 1.280025e-67 | 1.252748e-65 | 0 |
2 | chr2_93645657_93648162 | 5.189615 | 2.360560e-06 | 4.324934e-05 | Alx4 | -10.292504 | 5.458739e-18 | 1.081356e-16 | 0 |
3 | chr3_33140350_33142508 | 5.534632 | 6.455627e-08 | 1.724615e-06 | Pex5l | -7.590236 | 2.351122e-24 | 6.841972e-23 | 0 |
4 | chr3_126498429_126499612 | 5.337659 | 5.594815e-07 | 1.202787e-05 | Arsj | -6.281259 | 8.667831e-27 | 2.783525e-25 | -58056 |
5 | chr3_126657669_126660188 | -5.397757 | 5.546104e-07 | 1.202787e-05 | Arsj | -6.281259 | 8.667831e-27 | 2.783525e-25 | -217296 |
6 | chr3_132085669_132092999 | 6.121213 | 1.509244e-105 | 2.850264e-102 | Dkk2 | -6.916087 | 9.389039e-178 | 5.513378e-175 | 0 |
7 | chr3_132093815_132106295 | 5.072335 | 9.590057e-65 | 6.924867e-62 | Dkk2 | -6.916087 | 9.389039e-178 | 5.513378e-175 | 0 |
8 | chr3_141699368_141702608 | 6.065565 | 2.403341e-29 | 5.413249e-27 | Bmpr1b | -5.570116 | 6.893076e-40 | 3.655998e-38 | 134528 |
9 | chr4_68535893_68538867 | 5.172571 | 1.974087e-15 | 1.648497e-13 | Brinp1 | -7.758307 | 5.384595e-10 | 5.726618e-09 | 222505 |
10 | chr4_68545353_68551019 | 6.825275 | 1.516093e-18 | 1.684235e-16 | Brinp1 | -7.758307 | 5.384595e-10 | 5.726618e-09 | 210353 |
11 | chr4_118022206_118024522 | -5.332271 | 1.094775e-06 | 2.210347e-05 | Artn | 9.083650 | 8.043160e-84 | 1.066497e-81 | -92444 |
12 | chr5_13001869_13003460 | 5.957213 | 2.037009e-10 | 8.898685e-09 | Sema3a | -5.771471 | 3.017136e-297 | 6.200968e-294 | 393324 |
13 | chr5_13276479_13283560 | 7.727895 | 3.536779e-34 | 1.033708e-31 | Sema3a | -5.771471 | 3.017136e-297 | 6.200968e-294 | 113224 |
14 | chr5_17080302_17082934 | 5.024623 | 9.959617e-06 | 1.561421e-04 | Hgf | -7.513756 | 2.543270e-09 | 2.523623e-08 | -460864 |
15 | chr5_28465271_28467276 | -5.397757 | 5.546104e-07 | 1.202787e-05 | Shh | 5.565109 | 2.158809e-156 | 9.593279e-154 | 0 |
16 | chr5_38081825_38086331 | 6.088429 | 2.350343e-11 | 1.192216e-09 | Nsg1 | -5.086635 | 2.233941e-111 | 4.963574e-109 | 50862 |
17 | chr7_56523378_56528436 | 6.721266 | 2.699280e-17 | 2.609056e-15 | Gabrg3 | -5.960560 | 2.341610e-05 | 1.323050e-04 | 195806 |
18 | chr7_111875691_111878313 | 5.109476 | 4.848742e-06 | 8.221096e-05 | Galnt18 | -5.959958 | 2.253335e-68 | 2.218523e-66 | -95715 |
19 | chr8_82329336_82331284 | 5.189615 | 2.360560e-06 | 4.324934e-05 | Il15 | -5.185933 | 8.096707e-04 | 3.267016e-03 | 340 |
20 | chr8_102781569_102784308 | 5.761340 | 3.626346e-09 | 1.222945e-07 | Cdh11 | -6.729272 | 1.932941e-232 | 1.986339e-229 | 0 |
21 | chr11_96341058_96343216 | 5.337659 | 5.594815e-07 | 1.202787e-05 | Hoxb3 | -6.433657 | 6.669080e-07 | 4.796719e-06 | 0 |
22 | chr11_96354206_96355627 | 5.109476 | 4.848742e-06 | 8.221096e-05 | Hoxb3 | -6.433657 | 6.669080e-07 | 4.796719e-06 | -6277 |
23 | chr11_96354206_96355627 | 5.109476 | 4.848742e-06 | 8.221096e-05 | Hoxb2 | -6.351656 | 3.359549e-06 | 2.173857e-05 | -193 |
24 | chr12_56506841_56514912 | -9.259195 | 2.083740e-95 | 3.009288e-92 | Nkx2-1 | 9.134857 | 1.061953e-60 | 9.189803e-59 | 17023 |
25 | chr13_117409406_117414817 | -5.127606 | 3.397970e-15 | 2.771547e-13 | Emb | -6.021510 | 3.917980e-06 | 2.506593e-05 | -134992 |
26 | chr14_66104432_66107303 | 6.455571 | 1.757505e-14 | 1.344190e-12 | Ephx2 | -6.872942 | 1.597944e-07 | 1.247549e-06 | 0 |
27 | chr14_88460573_88463273 | 5.594736 | 3.142836e-08 | 8.899628e-07 | Pcdh20 | -6.097424 | 1.179837e-05 | 6.988068e-05 | 1474 |
28 | chr14_88898125_88900211 | -5.263671 | 2.162151e-06 | 4.039800e-05 | Pcdh20 | -6.097424 | 1.179837e-05 | 6.988068e-05 | -426730 |
29 | chr15_11692586_11694713 | 6.046002 | 4.827833e-11 | 2.333231e-09 | Npr3 | -8.356128 | 2.957230e-242 | 3.473055e-239 | 145183 |
30 | chr15_11743634_11745456 | 6.922288 | 8.515240e-20 | 1.034939e-17 | Npr3 | -8.356128 | 2.957230e-242 | 3.473055e-239 | 94440 |
31 | chr15_11782997_11784930 | 5.957213 | 2.037009e-10 | 8.898685e-09 | Npr3 | -8.356128 | 2.957230e-242 | 3.473055e-239 | 54966 |
32 | chr18_53465649_53470088 | 5.761340 | 3.626346e-09 | 1.222945e-07 | Prdm6 | -9.029699 | 4.482978e-14 | 6.960257e-13 | 0 |
33 | chr19_59159949_59161669 | -6.094880 | 4.260481e-11 | 2.067175e-09 | Vax1 | 7.636277 | 8.070915e-31 | 3.071805e-29 | 2816 |
34 | chr19_59163812_59165938 | -5.788022 | 4.812885e-09 | 1.603272e-07 | Vax1 | 7.636277 | 8.070915e-31 | 3.071805e-29 | 0 |
35 | chrX_93281556_93288401 | 5.093695 | 9.655833e-32 | 2.469379e-29 | Arx | -5.380834 | 6.537245e-123 | 1.853196e-120 | 0 |
36 | chrY_1009018_1011799 | -5.686862 | 1.864054e-08 | 5.507147e-07 | Eif2s3y | 11.645099 | 9.414457e-23 | 2.468780e-21 | 0 |
37 | chrY_1243715_1246316 | -5.520433 | 1.425488e-07 | 3.468498e-06 | Ddx3y | 11.525927 | 2.291430e-22 | 5.823136e-21 | 14399 |
38 | chrY_1243715_1246316 | -5.520433 | 1.425488e-07 | 3.468498e-06 | Uty | 8.369064 | 1.224906e-22 | 3.191744e-21 | 0 |
39 | chrY_1282482_1287504 | -5.191646 | 4.272455e-06 | 7.350598e-05 | Ddx3y | 11.525927 | 2.291430e-22 | 5.823136e-21 | 0 |
Next, we visualize, for specific peaks, the magnitude of differential binding and the expression of nearby genes using the dotplot function. This function creates a dotplot with three columns (below). The first shows the relative binding of Brd4 at a chromsomal locus in males and females, the second and third columns show the expression levels of the nearest and second nearest genes in males and females.
[27]:
cc.pl.dotplot_bulk(adata_cc, rna, selected_list = list(np.unique(np.array(result["Peak"]))), num_list = [3,3], dotsize = 500,
cmap = 'plasma', figsize = (16, 16), sort_by_chrom = True, topspace = 0.96)
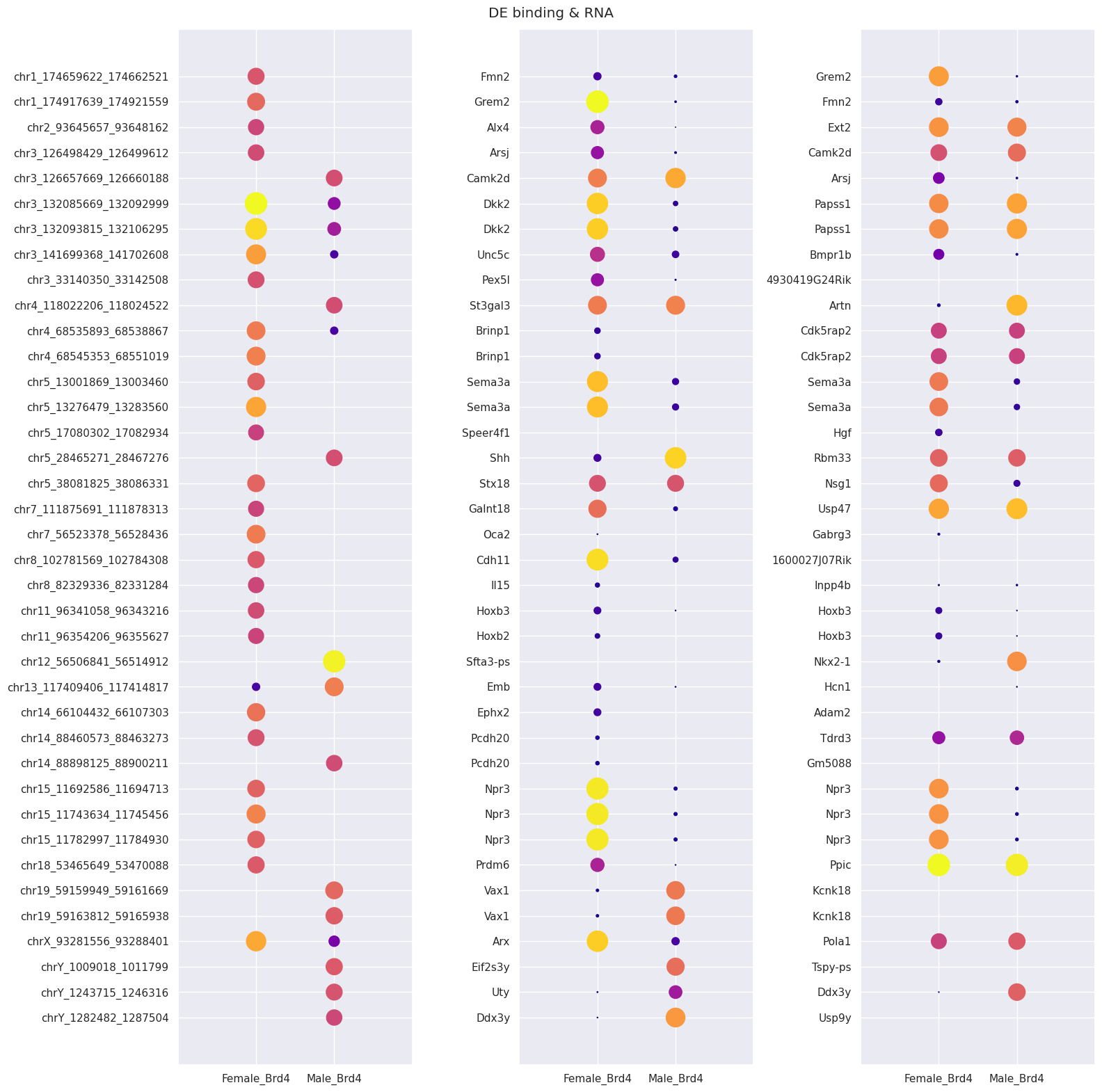
We can see from these results that all of the peaks visualized here display differential binding, and either the nearest, or second nearest gene has differential expression. In most cases, increased Brd4 binding is associated with an increase in gene expression.
Pycallingcards provides functionality to explore relatinoships between differentially bound regions and nearby GWAS identified SNPs. Since the data analyzed here were collected in murine cells, we must first lift over any interesting peaks to the human genome before we can look for nearby disease associated SNPs. We use liftover to get the resuls.
[28]:
result = cc.tl.result_mapping(result)
result
Start mapping the peaks to the new genome.
100%|██████████| 40/40 [00:00<00:00, 69.43it/s]
Start finding location of genes in the new genome.
100%|██████████| 40/40 [00:00<00:00, 287.78it/s]
[28]:
Peak | logfoldchanges_peak | Pvalue_peak | Pvalue_adj_peak | Gene | Score_gene | Pvalue_gene | Pvalue_adj_gene | Distance_peak_to_gene | Chr_liftover | Start_liftover | End_liftover | Chr_hg38 | Start_hg38 | End_hg38 | |
---|---|---|---|---|---|---|---|---|---|---|---|---|---|---|---|
0 | chr1_174659622_174662521 | 5.652435 | 1.530047e-08 | 4.586591e-07 | Grem2 | -10.401831 | 1.280025e-67 | 1.252748e-65 | 171264 | chr1 | 240280756 | 240283835 | chr1 | 240489572 | 240612162 |
1 | chr1_174917639_174921559 | 6.208699 | 2.711848e-12 | 1.562878e-10 | Grem2 | -10.401831 | 1.280025e-67 | 1.252748e-65 | 0 | chr1 | 240608636 | 240611870 | chr1 | 240489572 | 240612162 |
2 | chr2_93645657_93648162 | 5.189615 | 2.360560e-06 | 4.324934e-05 | Alx4 | -10.292504 | 5.458739e-18 | 1.081356e-16 | 0 | chr11 | 44303696 | 44306415 | chr11 | 44260727 | 44310166 |
3 | chr3_33140350_33142508 | 5.534632 | 6.455627e-08 | 1.724615e-06 | Pex5l | -7.590236 | 2.351122e-24 | 6.841972e-23 | 0 | chr3 | 180033883 | 180036186 | chr3 | 179794958 | 180037053 |
4 | chr3_126498429_126499612 | 5.337659 | 5.594815e-07 | 1.202787e-05 | Arsj | -6.281259 | 8.667831e-27 | 2.783525e-25 | -58056 | chr4 | 113839066 | 113844568 | chr4 | 113900283 | 113979722 |
5 | chr3_126657669_126660188 | -5.397757 | 5.546104e-07 | 1.202787e-05 | Arsj | -6.281259 | 8.667831e-27 | 2.783525e-25 | -217296 | chr4 | 113683844 | 113686758 | chr4 | 113900283 | 113979722 |
6 | chr3_132085669_132092999 | 6.121213 | 1.509244e-105 | 2.850264e-102 | Dkk2 | -6.916087 | 9.389039e-178 | 5.513378e-175 | 0 | chr4 | 107029387 | 107035946 | chr4 | 106921801 | 107036296 |
7 | chr3_132093815_132106295 | 5.072335 | 9.590057e-65 | 6.924867e-62 | Dkk2 | -6.916087 | 9.389039e-178 | 5.513378e-175 | 0 | chr4 | 107015456 | 107028826 | chr4 | 106921801 | 107036296 |
8 | chr3_141699368_141702608 | 6.065565 | 2.403341e-29 | 5.413249e-27 | Bmpr1b | -5.570116 | 6.893076e-40 | 3.655998e-38 | 134528 | chr4 | 95306647 | 95309431 | chr4 | 94757976 | 95158450 |
9 | chr4_68535893_68538867 | 5.172571 | 1.974087e-15 | 1.648497e-13 | Brinp1 | -7.758307 | 5.384595e-10 | 5.726618e-09 | 222505 | chr9 | 118993297 | 118996641 | chr9 | 119166629 | 119369461 |
10 | chr4_68545353_68551019 | 6.825275 | 1.516093e-18 | 1.684235e-16 | Brinp1 | -7.758307 | 5.384595e-10 | 5.726618e-09 | 210353 | chr9 | 119002823 | 119008509 | chr9 | 119166629 | 119369461 |
11 | chr4_118022206_118024522 | -5.332271 | 1.094775e-06 | 2.210347e-05 | Artn | 9.083650 | 8.043160e-84 | 1.066497e-81 | -92444 | chr1 | 43824383 | 43825212 | chr1 | 43933319 | 43937240 |
12 | chr5_13001869_13003460 | 5.957213 | 2.037009e-10 | 8.898685e-09 | Sema3a | -5.771471 | 3.017136e-297 | 6.200968e-294 | 393324 | chr7 | 83958342 | 84194901 | |||
13 | chr5_13276479_13283560 | 7.727895 | 3.536779e-34 | 1.033708e-31 | Sema3a | -5.771471 | 3.017136e-297 | 6.200968e-294 | 113224 | chr7 | 84314176 | 84318280 | chr7 | 83958342 | 84194901 |
14 | chr5_17080302_17082934 | 5.024623 | 9.959617e-06 | 1.561421e-04 | Hgf | -7.513756 | 2.543270e-09 | 2.523623e-08 | -460864 | chr7 | 81335127 | 81335733 | chr7 | 81699007 | 81770198 |
15 | chr5_28465271_28467276 | -5.397757 | 5.546104e-07 | 1.202787e-05 | Shh | 5.565109 | 2.158809e-156 | 9.593279e-154 | 0 | chr7 | 155810616 | 155812624 | chr7 | 155799983 | 155812273 |
16 | chr5_38081825_38086331 | 6.088429 | 2.350343e-11 | 1.192216e-09 | Nsg1 | -5.086635 | 2.233941e-111 | 4.963574e-109 | 50862 | chr4 | 4488485 | 4493470 | chr4 | 4386255 | 4419058 |
17 | chr7_56523378_56528436 | 6.721266 | 2.699280e-17 | 2.609056e-15 | Gabrg3 | -5.960560 | 2.341610e-05 | 1.323050e-04 | 195806 | chr15 | 26971281 | 27533227 | |||
18 | chr7_111875691_111878313 | 5.109476 | 4.848742e-06 | 8.221096e-05 | Galnt18 | -5.959958 | 2.253335e-68 | 2.218523e-66 | -95715 | chr11 | 11723801 | 11725419 | chr11 | 11270873 | 11622014 |
19 | chr8_82329336_82331284 | 5.189615 | 2.360560e-06 | 4.324934e-05 | Il15 | -5.185933 | 8.096707e-04 | 3.267016e-03 | 340 | chr4 | 141733679 | 141736013 | chr4 | 141636595 | 141733987 |
20 | chr8_102781569_102784308 | 5.761340 | 3.626346e-09 | 1.222945e-07 | Cdh11 | -6.729272 | 1.932941e-232 | 1.986339e-229 | 0 | chr16 | 65118621 | 65121296 | chr16 | 64943752 | 65122137 |
21 | chr11_96341058_96343216 | 5.337659 | 5.594815e-07 | 1.202787e-05 | Hoxb3 | -6.433657 | 6.669080e-07 | 4.796719e-06 | 0 | chr17 | 48553538 | 48555622 | chr17 | 48548869 | 48582622 |
22 | chr11_96354206_96355627 | 5.109476 | 4.848742e-06 | 8.221096e-05 | Hoxb3 | -6.433657 | 6.669080e-07 | 4.796719e-06 | -6277 | chr17 | 48540790 | 48542479 | chr17 | 48548869 | 48582622 |
23 | chr11_96354206_96355627 | 5.109476 | 4.848742e-06 | 8.221096e-05 | Hoxb2 | -6.351656 | 3.359549e-06 | 2.173857e-05 | -193 | chr17 | 48540790 | 48542479 | chr17 | 48542655 | 48545031 |
24 | chr12_56506841_56514912 | -9.259195 | 2.083740e-95 | 3.009288e-92 | Nkx2-1 | 9.134857 | 1.061953e-60 | 9.189803e-59 | 17023 | chr14 | 36491565 | 36499712 | chr14 | 36516398 | 36520225 |
25 | chr13_117409406_117414817 | -5.127606 | 3.397970e-15 | 2.771547e-13 | Emb | -6.021510 | 3.917980e-06 | 2.506593e-05 | -134992 | chr5 | 50396196 | 50441400 | |||
26 | chr14_66104432_66107303 | 6.455571 | 1.757505e-14 | 1.344190e-12 | Ephx2 | -6.872942 | 1.597944e-07 | 1.247549e-06 | 0 | chr8 | 27506903 | 27511135 | chr8 | 27491001 | 27544922 |
27 | chr14_88460573_88463273 | 5.594736 | 3.142836e-08 | 8.899628e-07 | Pcdh20 | -6.097424 | 1.179837e-05 | 6.988068e-05 | 1474 | chr13 | 61406547 | 61407676 | chr13 | 61409685 | 61415522 |
28 | chr14_88898125_88900211 | -5.263671 | 2.162151e-06 | 4.039800e-05 | Pcdh20 | -6.097424 | 1.179837e-05 | 6.988068e-05 | -426730 | chr13 | 61862674 | 61862983 | chr13 | 61409685 | 61415522 |
29 | chr15_11692586_11694713 | 6.046002 | 4.827833e-11 | 2.333231e-09 | Npr3 | -8.356128 | 2.957230e-242 | 3.473055e-239 | 145183 | chr5 | 33023231 | 33024567 | chr5 | 32710636 | 32791724 |
30 | chr15_11743634_11745456 | 6.922288 | 8.515240e-20 | 1.034939e-17 | Npr3 | -8.356128 | 2.957230e-242 | 3.473055e-239 | 94440 | chr5 | 32929090 | 32932829 | chr5 | 32710636 | 32791724 |
31 | chr15_11782997_11784930 | 5.957213 | 2.037009e-10 | 8.898685e-09 | Npr3 | -8.356128 | 2.957230e-242 | 3.473055e-239 | 54966 | chr5 | 32864592 | 32866245 | chr5 | 32710636 | 32791724 |
32 | chr18_53465649_53470088 | 5.761340 | 3.626346e-09 | 1.222945e-07 | Prdm6 | -9.029699 | 4.482978e-14 | 6.960257e-13 | 0 | chr5 | 123091173 | 123096087 | chr5 | 123089102 | 123194266 |
33 | chr19_59159949_59161669 | -6.094880 | 4.260481e-11 | 2.067175e-09 | Vax1 | 7.636277 | 8.070915e-31 | 3.071805e-29 | 2816 | chr10 | 117127894 | 117129270 | chr10 | 117128520 | 117138301 |
34 | chr19_59163812_59165938 | -5.788022 | 4.812885e-09 | 1.603272e-07 | Vax1 | 7.636277 | 8.070915e-31 | 3.071805e-29 | 0 | chr10 | 117131583 | 117133776 | chr10 | 117128520 | 117138301 |
35 | chrX_93281556_93288401 | 5.093695 | 9.655833e-32 | 2.469379e-29 | Arx | -5.380834 | 6.537245e-123 | 1.853196e-120 | 0 | chrX | 25013967 | 25020980 | chrX | 25003695 | 25015948 |
36 | chrY_1009018_1011799 | -5.686862 | 1.864054e-08 | 5.507147e-07 | Eif2s3y | 11.645099 | 9.414457e-23 | 2.468780e-21 | 0 | chrX | 24054686 | 24057370 | |||
37 | chrY_1243715_1246316 | -5.520433 | 1.425488e-07 | 3.468498e-06 | Ddx3y | 11.525927 | 2.291430e-22 | 5.823136e-21 | 14399 | chrY | 13478375 | 13480067 | chrY | 12903998 | 12920478 |
38 | chrY_1243715_1246316 | -5.520433 | 1.425488e-07 | 3.468498e-06 | Uty | 8.369064 | 1.224906e-22 | 3.191744e-21 | 0 | chrY | 13478375 | 13480067 | chrY | 13248378 | 13480670 |
39 | chrY_1282482_1287504 | -5.191646 | 4.272455e-06 | 7.350598e-05 | Ddx3y | 11.525927 | 2.291430e-22 | 5.823136e-21 | 0 | chrY | 12903183 | 12909538 | chrY | 12903998 | 12920478 |
[29]:
GWAS_result = cc.tl.GWAS(result)
GWAS_result
[29]:
Peak | logfoldchanges_peak | Pvalue_peak | Pvalue_adj_peak | Gene | Score_gene | Pvalue_gene | Pvalue_adj_gene | Distance_peak_to_gene | Chr_liftover | Start_liftover | End_liftover | Chr_hg38 | Start_hg38 | End_hg38 | GWAS | |
---|---|---|---|---|---|---|---|---|---|---|---|---|---|---|---|---|
0 | chr1_174659622_174662521 | 5.652435 | 1.530047e-08 | 4.586591e-07 | Grem2 | -10.401831 | 1.280025e-67 | 1.252748e-65 | 171264 | chr1 | 240280756 | 240283835 | chr1 | 240489572 | 240612162 | Coronary heart disease |
1 | chr1_174917639_174921559 | 6.208699 | 2.711848e-12 | 1.562878e-10 | Grem2 | -10.401831 | 1.280025e-67 | 1.252748e-65 | 0 | chr1 | 240608636 | 240611870 | chr1 | 240489572 | 240612162 | |
2 | chr2_93645657_93648162 | 5.189615 | 2.360560e-06 | 4.324934e-05 | Alx4 | -10.292504 | 5.458739e-18 | 1.081356e-16 | 0 | chr11 | 44303696 | 44306415 | chr11 | 44260727 | 44310166 | |
3 | chr3_33140350_33142508 | 5.534632 | 6.455627e-08 | 1.724615e-06 | Pex5l | -7.590236 | 2.351122e-24 | 6.841972e-23 | 0 | chr3 | 180033883 | 180036186 | chr3 | 179794958 | 180037053 | |
4 | chr3_126498429_126499612 | 5.337659 | 5.594815e-07 | 1.202787e-05 | Arsj | -6.281259 | 8.667831e-27 | 2.783525e-25 | -58056 | chr4 | 113839066 | 113844568 | chr4 | 113900283 | 113979722 | |
5 | chr3_126657669_126660188 | -5.397757 | 5.546104e-07 | 1.202787e-05 | Arsj | -6.281259 | 8.667831e-27 | 2.783525e-25 | -217296 | chr4 | 113683844 | 113686758 | chr4 | 113900283 | 113979722 | |
6 | chr3_132085669_132092999 | 6.121213 | 1.509244e-105 | 2.850264e-102 | Dkk2 | -6.916087 | 9.389039e-178 | 5.513378e-175 | 0 | chr4 | 107029387 | 107035946 | chr4 | 106921801 | 107036296 | |
7 | chr3_132093815_132106295 | 5.072335 | 9.590057e-65 | 6.924867e-62 | Dkk2 | -6.916087 | 9.389039e-178 | 5.513378e-175 | 0 | chr4 | 107015456 | 107028826 | chr4 | 106921801 | 107036296 | |
8 | chr3_141699368_141702608 | 6.065565 | 2.403341e-29 | 5.413249e-27 | Bmpr1b | -5.570116 | 6.893076e-40 | 3.655998e-38 | 134528 | chr4 | 95306647 | 95309431 | chr4 | 94757976 | 95158450 | |
9 | chr4_68535893_68538867 | 5.172571 | 1.974087e-15 | 1.648497e-13 | Brinp1 | -7.758307 | 5.384595e-10 | 5.726618e-09 | 222505 | chr9 | 118993297 | 118996641 | chr9 | 119166629 | 119369461 | Gut microbiota (bacterial taxa, hurdle binary ... |
10 | chr4_68545353_68551019 | 6.825275 | 1.516093e-18 | 1.684235e-16 | Brinp1 | -7.758307 | 5.384595e-10 | 5.726618e-09 | 210353 | chr9 | 119002823 | 119008509 | chr9 | 119166629 | 119369461 | |
11 | chr4_118022206_118024522 | -5.332271 | 1.094775e-06 | 2.210347e-05 | Artn | 9.083650 | 8.043160e-84 | 1.066497e-81 | -92444 | chr1 | 43824383 | 43825212 | chr1 | 43933319 | 43937240 | |
12 | chr5_13001869_13003460 | 5.957213 | 2.037009e-10 | 8.898685e-09 | Sema3a | -5.771471 | 3.017136e-297 | 6.200968e-294 | 393324 | chr7 | 83958342 | 84194901 | ||||
13 | chr5_13276479_13283560 | 7.727895 | 3.536779e-34 | 1.033708e-31 | Sema3a | -5.771471 | 3.017136e-297 | 6.200968e-294 | 113224 | chr7 | 84314176 | 84318280 | chr7 | 83958342 | 84194901 | |
14 | chr5_17080302_17082934 | 5.024623 | 9.959617e-06 | 1.561421e-04 | Hgf | -7.513756 | 2.543270e-09 | 2.523623e-08 | -460864 | chr7 | 81335127 | 81335733 | chr7 | 81699007 | 81770198 | |
15 | chr5_28465271_28467276 | -5.397757 | 5.546104e-07 | 1.202787e-05 | Shh | 5.565109 | 2.158809e-156 | 9.593279e-154 | 0 | chr7 | 155810616 | 155812624 | chr7 | 155799983 | 155812273 | Urate levels |
16 | chr5_38081825_38086331 | 6.088429 | 2.350343e-11 | 1.192216e-09 | Nsg1 | -5.086635 | 2.233941e-111 | 4.963574e-109 | 50862 | chr4 | 4488485 | 4493470 | chr4 | 4386255 | 4419058 | |
17 | chr7_56523378_56528436 | 6.721266 | 2.699280e-17 | 2.609056e-15 | Gabrg3 | -5.960560 | 2.341610e-05 | 1.323050e-04 | 195806 | chr15 | 26971281 | 27533227 | ||||
18 | chr7_111875691_111878313 | 5.109476 | 4.848742e-06 | 8.221096e-05 | Galnt18 | -5.959958 | 2.253335e-68 | 2.218523e-66 | -95715 | chr11 | 11723801 | 11725419 | chr11 | 11270873 | 11622014 | |
19 | chr8_82329336_82331284 | 5.189615 | 2.360560e-06 | 4.324934e-05 | Il15 | -5.185933 | 8.096707e-04 | 3.267016e-03 | 340 | chr4 | 141733679 | 141736013 | chr4 | 141636595 | 141733987 | |
20 | chr8_102781569_102784308 | 5.761340 | 3.626346e-09 | 1.222945e-07 | Cdh11 | -6.729272 | 1.932941e-232 | 1.986339e-229 | 0 | chr16 | 65118621 | 65121296 | chr16 | 64943752 | 65122137 | |
21 | chr11_96341058_96343216 | 5.337659 | 5.594815e-07 | 1.202787e-05 | Hoxb3 | -6.433657 | 6.669080e-07 | 4.796719e-06 | 0 | chr17 | 48553538 | 48555622 | chr17 | 48548869 | 48582622 | Migraine; Insomnia |
22 | chr11_96354206_96355627 | 5.109476 | 4.848742e-06 | 8.221096e-05 | Hoxb3 | -6.433657 | 6.669080e-07 | 4.796719e-06 | -6277 | chr17 | 48540790 | 48542479 | chr17 | 48548869 | 48582622 | Cystatin C levels |
23 | chr11_96354206_96355627 | 5.109476 | 4.848742e-06 | 8.221096e-05 | Hoxb2 | -6.351656 | 3.359549e-06 | 2.173857e-05 | -193 | chr17 | 48540790 | 48542479 | chr17 | 48542655 | 48545031 | Cystatin C levels |
24 | chr12_56506841_56514912 | -9.259195 | 2.083740e-95 | 3.009288e-92 | Nkx2-1 | 9.134857 | 1.061953e-60 | 9.189803e-59 | 17023 | chr14 | 36491565 | 36499712 | chr14 | 36516398 | 36520225 | Nonsyndromic orofacial cleft x sex interaction... |
25 | chr13_117409406_117414817 | -5.127606 | 3.397970e-15 | 2.771547e-13 | Emb | -6.021510 | 3.917980e-06 | 2.506593e-05 | -134992 | chr5 | 50396196 | 50441400 | ||||
26 | chr14_66104432_66107303 | 6.455571 | 1.757505e-14 | 1.344190e-12 | Ephx2 | -6.872942 | 1.597944e-07 | 1.247549e-06 | 0 | chr8 | 27506903 | 27511135 | chr8 | 27491001 | 27544922 | Childhood ear infection |
27 | chr14_88460573_88463273 | 5.594736 | 3.142836e-08 | 8.899628e-07 | Pcdh20 | -6.097424 | 1.179837e-05 | 6.988068e-05 | 1474 | chr13 | 61406547 | 61407676 | chr13 | 61409685 | 61415522 | |
28 | chr14_88898125_88900211 | -5.263671 | 2.162151e-06 | 4.039800e-05 | Pcdh20 | -6.097424 | 1.179837e-05 | 6.988068e-05 | -426730 | chr13 | 61862674 | 61862983 | chr13 | 61409685 | 61415522 | |
29 | chr15_11692586_11694713 | 6.046002 | 4.827833e-11 | 2.333231e-09 | Npr3 | -8.356128 | 2.957230e-242 | 3.473055e-239 | 145183 | chr5 | 33023231 | 33024567 | chr5 | 32710636 | 32791724 | |
30 | chr15_11743634_11745456 | 6.922288 | 8.515240e-20 | 1.034939e-17 | Npr3 | -8.356128 | 2.957230e-242 | 3.473055e-239 | 94440 | chr5 | 32929090 | 32932829 | chr5 | 32710636 | 32791724 | |
31 | chr15_11782997_11784930 | 5.957213 | 2.037009e-10 | 8.898685e-09 | Npr3 | -8.356128 | 2.957230e-242 | 3.473055e-239 | 54966 | chr5 | 32864592 | 32866245 | chr5 | 32710636 | 32791724 | |
32 | chr18_53465649_53470088 | 5.761340 | 3.626346e-09 | 1.222945e-07 | Prdm6 | -9.029699 | 4.482978e-14 | 6.960257e-13 | 0 | chr5 | 123091173 | 123096087 | chr5 | 123089102 | 123194266 | |
33 | chr19_59159949_59161669 | -6.094880 | 4.260481e-11 | 2.067175e-09 | Vax1 | 7.636277 | 8.070915e-31 | 3.071805e-29 | 2816 | chr10 | 117127894 | 117129270 | chr10 | 117128520 | 117138301 | |
34 | chr19_59163812_59165938 | -5.788022 | 4.812885e-09 | 1.603272e-07 | Vax1 | 7.636277 | 8.070915e-31 | 3.071805e-29 | 0 | chr10 | 117131583 | 117133776 | chr10 | 117128520 | 117138301 | Age at first birth; Nonsyndromic cleft lip wit... |
35 | chrX_93281556_93288401 | 5.093695 | 9.655833e-32 | 2.469379e-29 | Arx | -5.380834 | 6.537245e-123 | 1.853196e-120 | 0 | chrX | 25013967 | 25020980 | chrX | 25003695 | 25015948 | |
36 | chrY_1009018_1011799 | -5.686862 | 1.864054e-08 | 5.507147e-07 | Eif2s3y | 11.645099 | 9.414457e-23 | 2.468780e-21 | 0 | chrX | 24054686 | 24057370 | ||||
37 | chrY_1243715_1246316 | -5.520433 | 1.425488e-07 | 3.468498e-06 | Ddx3y | 11.525927 | 2.291430e-22 | 5.823136e-21 | 14399 | chrY | 13478375 | 13480067 | chrY | 12903998 | 12920478 | |
38 | chrY_1243715_1246316 | -5.520433 | 1.425488e-07 | 3.468498e-06 | Uty | 8.369064 | 1.224906e-22 | 3.191744e-21 | 0 | chrY | 13478375 | 13480067 | chrY | 13248378 | 13480670 | |
39 | chrY_1282482_1287504 | -5.191646 | 4.272455e-06 | 7.350598e-05 | Ddx3y | 11.525927 | 2.291430e-22 | 5.823136e-21 | 0 | chrY | 12903183 | 12909538 | chrY | 12903998 | 12920478 |
Save the file if needed.
[30]:
adata_cc.write("Brd4_bindings_bulk.h5ad")